Opening and transforming data with OpenRefine
OpenRefine originated as GoogleRefine. Google later open sourced the code. It is a great tool to sift through the data quickly, clean it, remove duplicate rows, analyze distributions or trends over time, and more.
In this and the following recipes, we will deal with the realEstate_trans_dirty.csv
file that is located in the Data/Chapter1
folder. The file has several issues that, over the course of the following recipes, we will see how to resolve.
First, when read from a text file, OpenRefine defaults the types of data to text; we will deal with data type transformations in this recipe. Otherwise, we will not be able to use facets to explore the numerical columns. Second, there are duplicates in the dataset (we will deal with them in the Remove duplicates recipe). Third, the city_state_zip
column, as the name suggests, is an amalgam of city, state, and zip. We prefer keeping these separate, and in the Using regular expressions and GREL to clean up data recipe, we will see how to extract such information. There is also some missing information about the sale price—we will impute the sale prices in the Imputing missing observations recipe.
Getting ready
To run through these examples, you need OpenRefine installed and running on your computer. You can download OpenRefine from http://openrefine.org/download.html. The installation instructions can be found at https://github.com/OpenRefine/OpenRefine/wiki/Installation-Instructions.
OpenRefine runs in a browser so you need an Internet browser installed on your computer. I tested it in Chrome and Safari and found no issues.
Note
The Mac OS X Yosemite comes with Java 8 installed by default. OpenRefine does not support it. You need to install Java 6 or 7—see https://support.apple.com/kb/DL1572?locale=en_US.
However, even after installing legacy versions of Java, I still experienced some issues with version 2.5 of OpenRefine on Mac OS X Yosemite and El Capitan. Using the beta version (2.6), even though it is still in development, worked fine.
No other prerequisites are required.
How to do it…
First, you need to start OpenRefine, open your browser, and type http://localhost:3333
. A window similar to the following screenshot should open:

The first thing you want to do is create a project. Click on Choose files, navigate to Data/Chapter1, and select realEstate_trans_dirty.csv. Click OK, then Next, and Create Project. After the data opens, you should see something similar to this:

Note that the beds, baths, sq__ft, price, latitude, and longitude data is treated as text and so is sale_date. While converting the former is easy, the format of sale_date is not as easy to play with in OpenRefine:
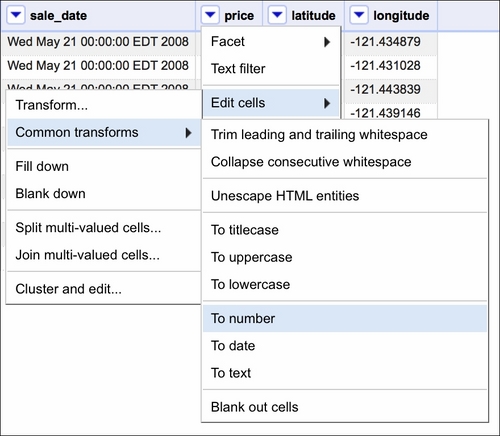
If the text data was in a format resembling, for example, 2008-05-21, we could just use the Google Refine Expression Language (GREL) method .toDate()
and OpenRefine would convert the dates for us. In our case, we need to use some trickery to convert the dates properly. First, we select a Transform option, as shown in the following screenshot:
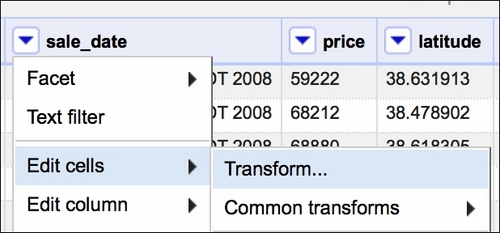
Then, in the window that opens, we will use GREL to convert the dates as follows:
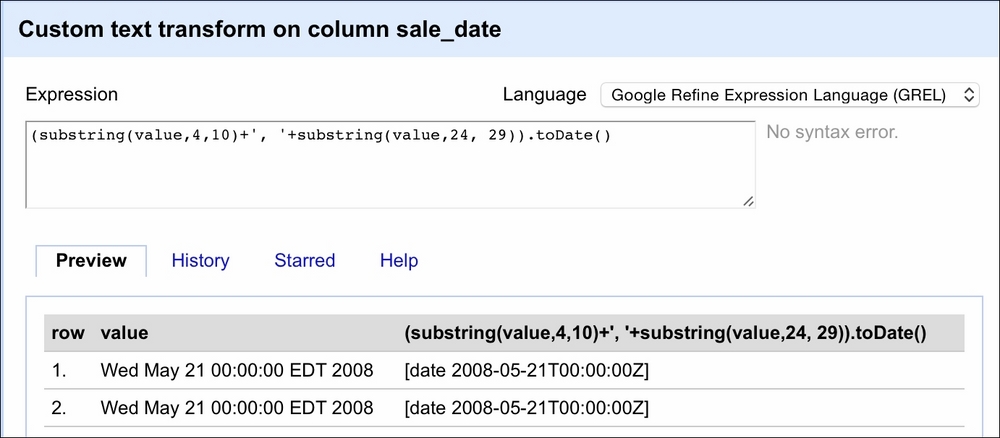
The value
variable here represents the value of each cell in the selected column (sale_date
). The first part of the expression extracts the month and day from the value, that is, we get in return May 21
by specifying that we want to retrieve a substring starting at the fourth character and finishing at the tenth character. The second substring(...)
method extracts the year from the string. We separate the two by a comma using the ...+', '+...
expression. The resulting value will result in the May 21, 2008
string pattern. Now OpenRefine can deal with this easily. Thus, we wrap our two substring
methods inside parentheses and use the .toDate()
method to convert the date properly. The Preview tab in the right column shows you the effect of our expression.
See also
A very good introduction and deep dives into the various aspects of OpenRefine can be found in the Using OpenRefine book by Ruben Verborgh and Max De Wilde at https://www.packtpub.com/big-data-and-business-intelligence/using-openrefine.