Now that we've defined and introduced healthcare analytics, it's important to give some background on the knowledge from which it draws. Healthcare analytics can be viewed as the intersection of three fields: healthcare (Healthcare Analytics), mathematics (Math), and computer science (CS), as seen in the following diagram. Let's explore each of these three areas in turn:
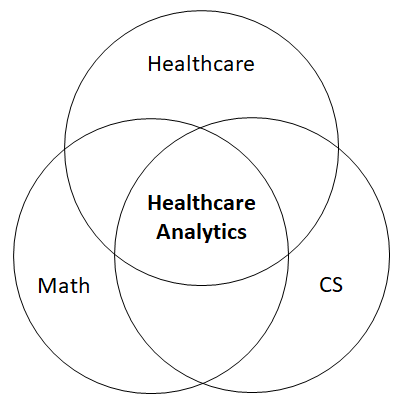