We can see that each feature in our observation seems to be on a different scale. Some values range in the hundreds, while others are between 1 and 12, or even binary. While neural networks may still ingest unscaled features, it almost exclusively prefers to deal with features on the same scale. In practice, a network can learn from heterogeneously scaled features, but it may take much longer to do so without any guarantee of finding an ideal minimum on the loss landscape. To allow our network to learn in an improved way for this dataset, we must homogenize our data through the process of feature-wise normalization. We can achieve this by subtracting the feature-specific mean and dividing it by the feature-specific standard deviation for each feature in our dataset. Note that in live-deployed models (for the stock exchange, for example), such a scaling...




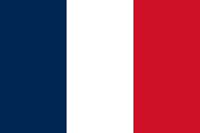


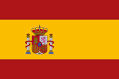




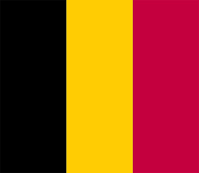
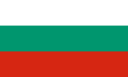
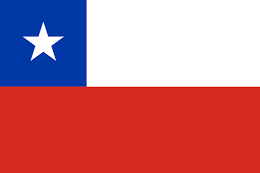
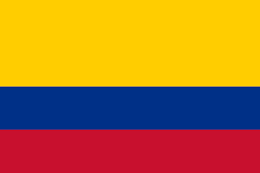
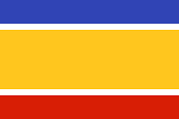

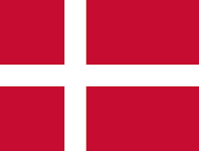



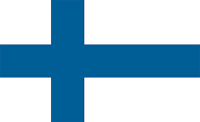

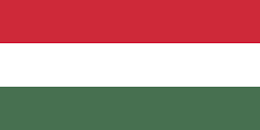






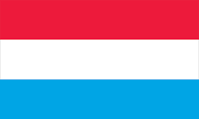
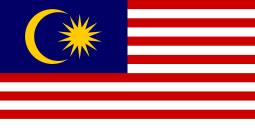

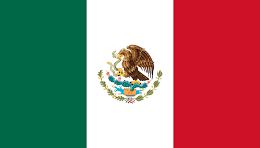

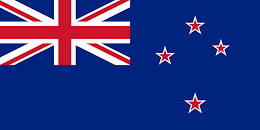







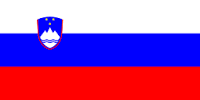




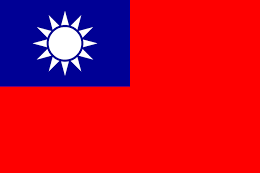


