As we saw in the last chapter, much of the computational work for ANNs involves calculating derivatives to find the gradient of the cost function. PyTorch uses the autograd package to perform automatic differentiation of operations on PyTorch tensors. To see how this works, let's look at an example:
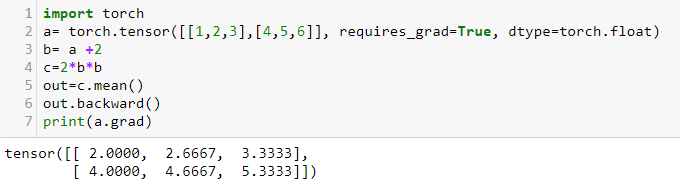
In the preceding code, we create a 2 x 3 torch tensor and, importantly, set the requires_grad attribute to True. This enables the calculation of gradients across subsequent operations. Notice also that we set the dtype to torch.float, since this is the data type that PyTorch uses for automatic differentiation. We perform a sequence of operations and then take the mean of the result. This returns a tensor containing a single scalar. This is normally what autograd requires to calculate the gradient of the preceding operations. This could be any sequence of operations;...