In the example of image classification that we covered in the last chapter, we split the data into two different halves, one for training and one for validation. It is a good practice to use a separate dataset to test the performance of your algorithm, as testing the algorithm on the training set may not give you the true generalization power of the algorithm. In most real-world use cases, based on the validation accuracy, we often tweak our algorithm in different ways, such as adding more layers or different layers, or using different techniques that we will cover in the later part of the chapter. So, there is a higher chance that your choices for tweaking the algorithm are based on the validation dataset. Algorithms trained this way tend to perform well in the training dataset and the validation dataset, but fail to generalize well on unseen...




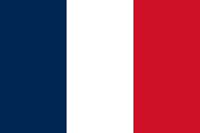


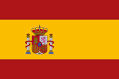



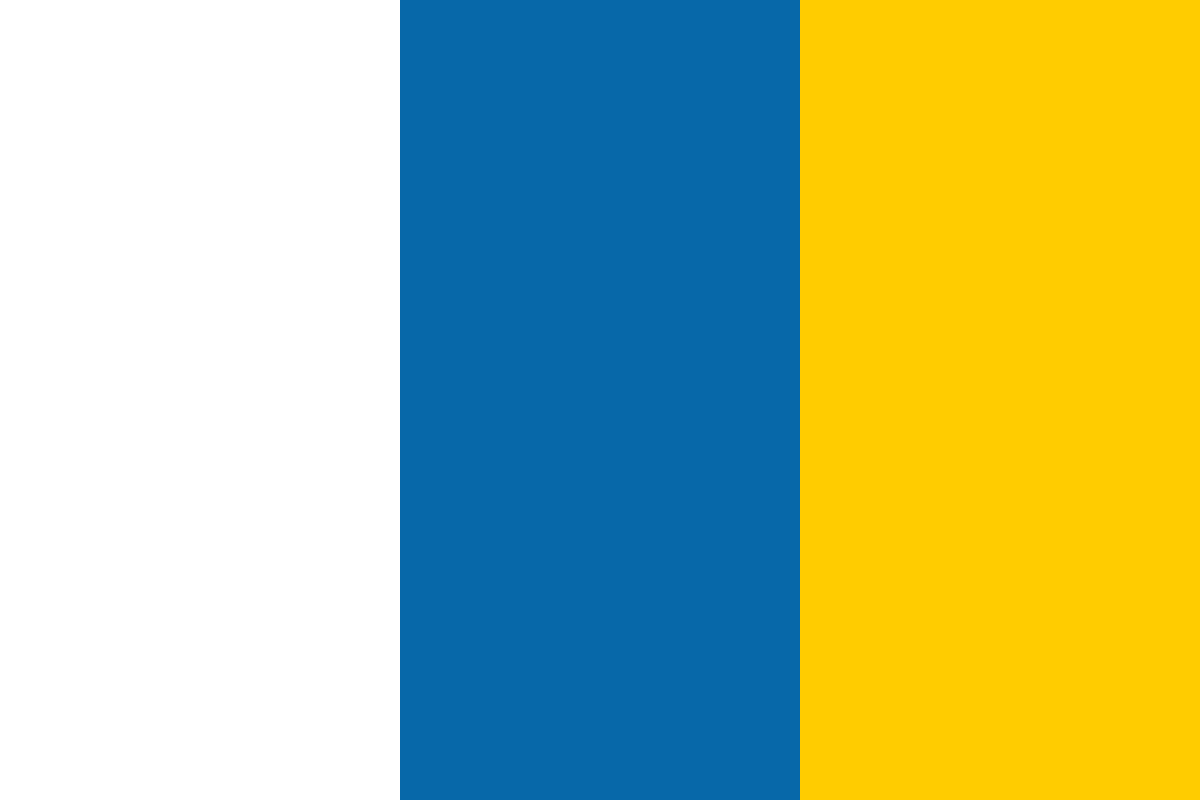
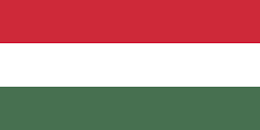

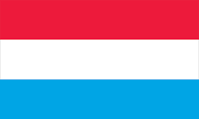





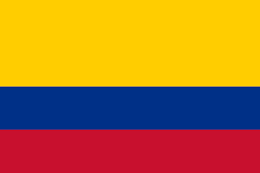
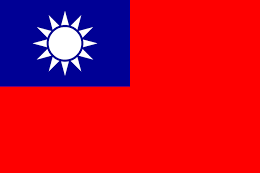
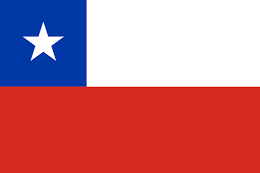



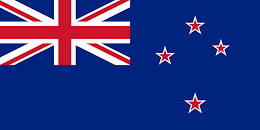
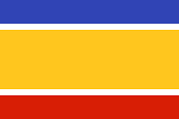
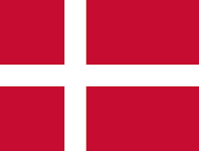
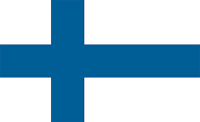







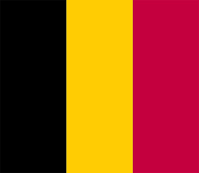

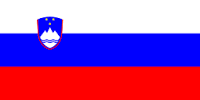





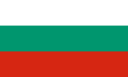


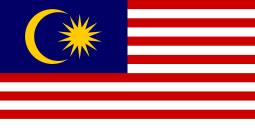



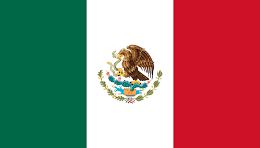
