Hierarchical linear regression
In the previous chapter, we learned the rudiments of hierarchical models. We can apply these concepts to linear regression and model several groups at the same time including estimations at the group level and estimations above the group level. As we saw, this is done by including hyperpriors.
We are going to create eight related data groups, including one with just one data point:
N = 20 M = 8 idx = np.repeat(range(M-1), N) idx = np.append(idx, 7) alpha_real = np.random.normal(2.5, 0.5, size=M) beta_real = np.random.beta(60, 10, size=M) eps_real = np.random.normal(0, 0.5, size=len(idx)) y_m = np.zeros(len(idx)) x_m = np.random.normal(10, 1, len(idx)) y_m = alpha_real[idx] + beta_real[idx] * x_m + eps_real
Our data looks like this:
j, k = 0, N for i in range(M): plt.subplot(2,4,i+1) plt.scatter(x_m[j:k], y_m[j:k]) plt.xlim(6, 15) plt.ylim(7, 17) j += N k += N plt.tight_layout()
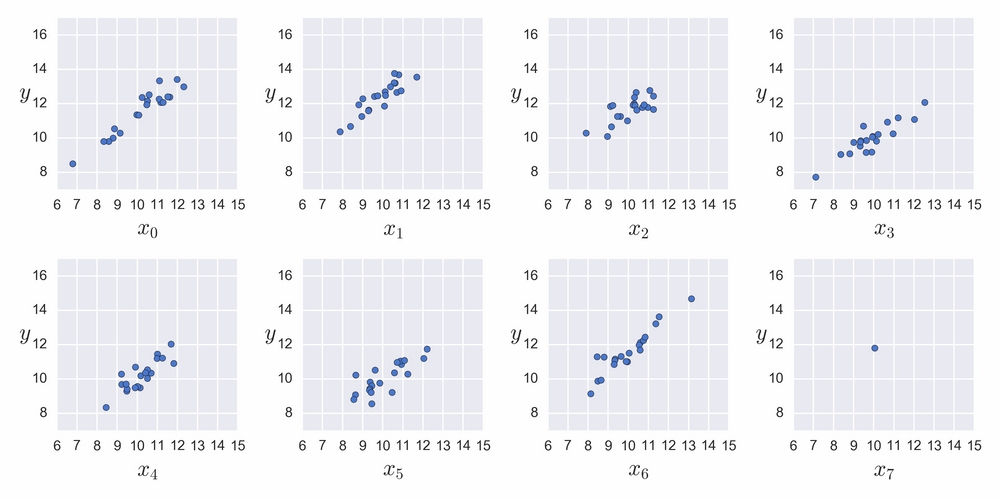
Now we are going to center the data before feeding it to...