Seq2seq model with attention
The summarization model has an Encoder part with a bidirectional RNN and a unidirectional decoder part. There is an attention layer that helps the Decoder focus on specific parts of the input while generating an output token. The overall architecture is shown in the following diagram:
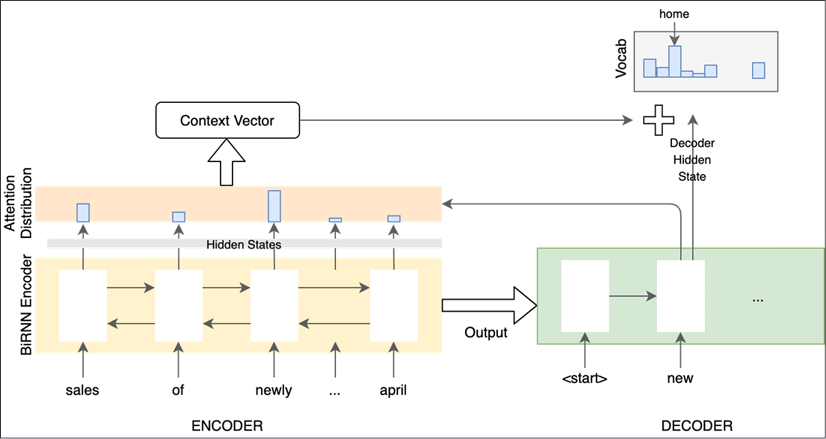
Figure 6.1: Seq2seq and attention model
These layers are detailed in the following subsections. All the code for these parts of the model are in the file seq2seq.py
. All the layers use common hyperparameters specified in the main function in the s2s-training.py
file:
embedding_dim = 128
units = 256 # from pointer generator paper
The code and architecture for this section have been inspired by the paper titled Get To The Point: Summarization with Pointer-Generator Networks by Abigail See, Peter Liu, and Chris Manning, published in April 2017. The fundamental architecture is easy to follow and provides impressive performance for a model that can be trained...