



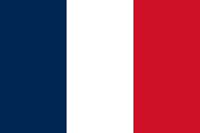


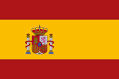




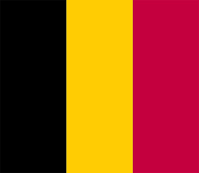
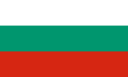
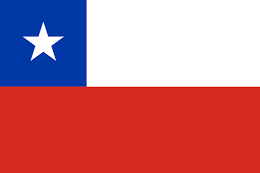
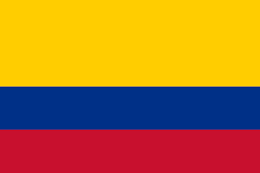
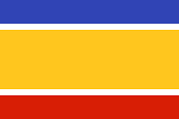

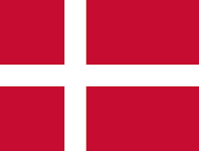



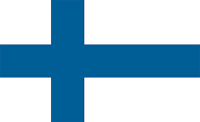

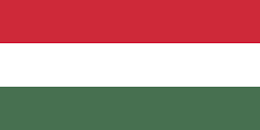






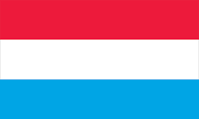
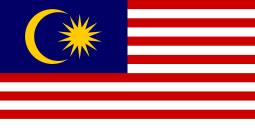

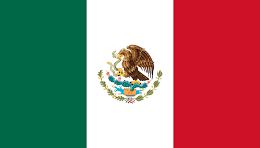

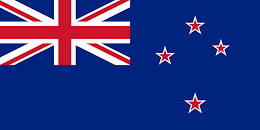







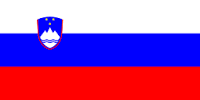




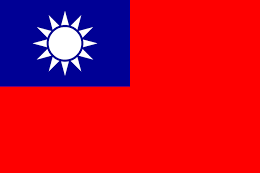



In this tutorial, we will introduce you to Machine learning agents in Unity that helps with AI game development. ML agents help in training intelligent agents within the game in a fun and informative way.
The ML-Agents SDK is useful in transforming games and simulations created using the Unity Editor into environments for training intelligent agents. These ML agents are trained using deep Reinforcement Learning, imitation learning, neuroevolution, or other machine learning methods via Python APIs.
Machine learning has a huge role to play in the development of AI games. From self-driving cars, playing Go and Chess, to computers being able to beat humans at classic Atari games, the advent of a group of technologies we colloquially call Machine Learning have come to dominate a new era in technological growth – a new era of growth that has been compared with the same importance as the discovery of electricity and has already been categorized as the next human technological age.
This tutorial is an excerpt taken from the book 'Learn Unity ML-Agents – Fundamentals of Unity Machine Learning' by Micheal Lanham.
So, let's get started!
Games and simulations are no stranger to AI technologies and there are numerous assets available to the Unity developer in order to provide simulated machine intelligence. These technologies include content like Behavior Trees, Finite State Machine, navigation meshes, A*, and other heuristic ways game developers use to simulate intelligence. So, why Machine Learning and why now?
The reason is due in large part to the OpenAI initiative, an initiative that encourages research across academia and the industry to share ideas and research on AI and ML. This has resulted in an explosion of growth in new ideas, methods, and areas for research. This means for games and simulations that we no longer have to fake or simulate intelligence. Now, we can build agents that learn from their environment and even learn to beat their human builders.
ML-Agents platform in Unity helps to build ML models that we can learn to play and simulate in various environments. Before we do that, let's first pull down the ML-Agents package from GitHub using git. Jump on your computer and open up a command prompt or shell window and follow along:
cd/
mkdir ML-Agents
cd ML-Agents git clone https://github.com/Unity-Technologies/ml-agents.git
cd ml-agents dir
Good—that should have been fairly painless. If you had issues pulling the code down, you can always visit the ML-Agents page on GitHub and manually pull the code down. Of course, we will be using more of git to manage and pull files, so you should resolve any problems you may have encountered.
Now that we have ML-Agents installed, we will take a look at one of Unity's sample projects that ship with a toolkit in the next section.
Unity ships the ML-Agents package with a number of prepared samples that demonstrate various aspects of learning and training scenarios. Let's open up Unity and load up a sample project and get a feel for how the ML-Agents run by following this exercise:
As you witnessed, the scene is currently set for the Player control, but obviously, we want to see how some of this ML-Agents stuff works. In order to do that, we need to change the Brain type that the agent is using. Follow along to switch the Brain type in the 3D Ball agent:
public float[] Decide( List<float> vectorObs, List<Texture2D> visualObs, float reward, bool done, List<float> memory) { if (gameObject.GetComponent<Brain() .brainParameters.vectorActionSpaceType == SpaceType.continuous) { List<float> act = new List<float>();
// state[5] is the velocity of the ball in the x orientation.
// We use this number to control the Platform's z axis rotation
speed,
// so that the Platform is tilted in the x orientation
correspondingly.
act.Add(vectorObs[5] * rotationSpeed);
// state[7] is the velocity of the ball in the z orientation.
// We use this number to control the Platform's x axis rotation
speed,
// so that the Platform is tilted in the z orientation
correspondingly.
act.Add(-vectorObs[7] * rotationSpeed);
return act.ToArray();
}
// If the vector action space type is discrete, then we don't do
anything.
return new float[1] { 1f };
}
Heuristic brains should not be confused with Internal brains. While you could replace the heuristic code in the 3D ball example with an ML algorithm, that is not the best practice for running an advanced ML such as Deep Learning algorithms.
We looked at the basics of machine learning in gaming and ML agents in Unity. This included shipping the ML-Agents package with a prepared sample that demonstrates various aspects of learning and training scenarios. We also looked at how to set up an agent brain.
If you found this post useful, be sure to check out the book 'Learn Unity ML-Agents – Fundamentals of Unity Machine Learning' to learn more other concepts such as creating an environment in Unity and Academy, Agent, and Brain objects in ML agents.
Implementing Unity game engine and assets for 2D game development [Tutorial]
Creating interactive Unity character animations and avatars [Tutorial]
Unity 2D & 3D game kits simplify Unity game development for beginners