Implementing a decision tree from scratch
We develop the CART tree algorithm by hand on a toy
dataset as follows:
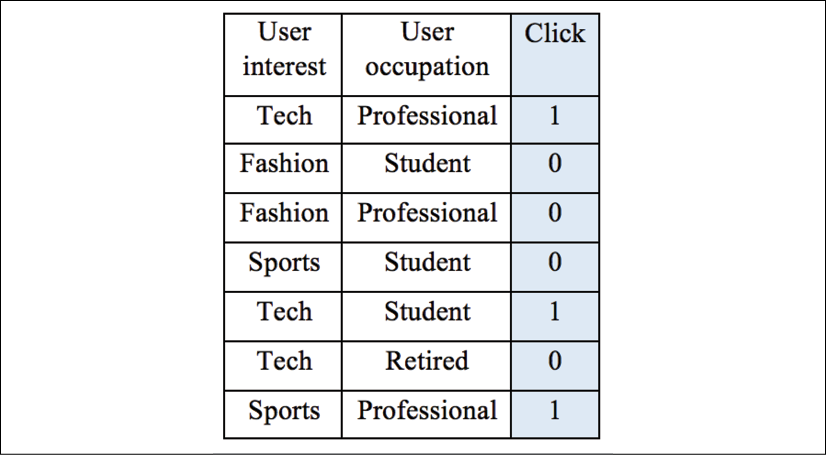
Figure 4.8: An example of ad data
To begin with, we decide on the first splitting point, the root, by trying out all possible values for each of the two features. We utilize the weighted_impurity
function we just defined to calculate the weighted Gini Impurity for each possible combination, as follows:
Gini(interest, tech) = weighted_impurity([[1, 1, 0],
[0, 0, 0, 1]]) = 0.405
Here, if we partition according to whether the user interest is tech, we have the 1st, 5th, and 6th samples for one group and the remaining samples for another group. Then the classes for the first group are [1, 1, 0]
, and the classes for the second group are [0, 0, 0, 1]
:
Gini(interest, Fashion) = weighted_impurity([[0, 0],
[1, 0, 1, 0, 1]]) = 0.343
Here, if we partition according to whether the user's interest is fashion, we have the 2nd and...