A decision tree is a simple yet powerful model for supervised learning problems. As the name suggests, we can think of it as a tree in which information flows along different branches—starting at the trunk and going all of the way to the individual leaves, making decisions about which branch to take at each junction.
This is basically a decision tree! Here is a simple example of a decision tree:
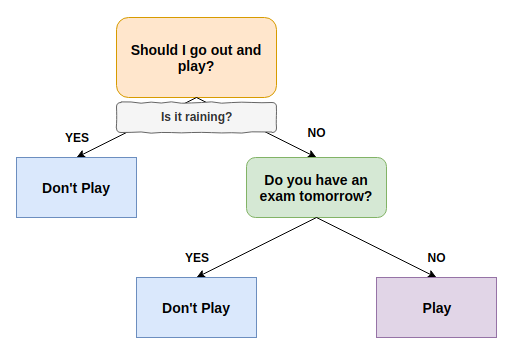
A decision tree is made of a hierarchy of questions or tests about the data (also known as decision nodes) and their possible consequences.
One of the true difficulties with building decision trees is how to pull out suitable features from the data. To make this clear, let's use a concrete example. Let's say we have a dataset consisting of a single email:
In [1]: data = [
... 'I am Mohammed Abacha, the son of the late Nigerian...