Fixing missing data in a dataset is the first important step for a lot of machine learning applications in healthcare, because we're often going to have missing data. There are different ways to handle this, and one of the easiest is to remove those rows entirely. This is especially the case if we're just trying to test a classification algorithm on a neural network, or train one for the first time. This is the route that we are going to take now:
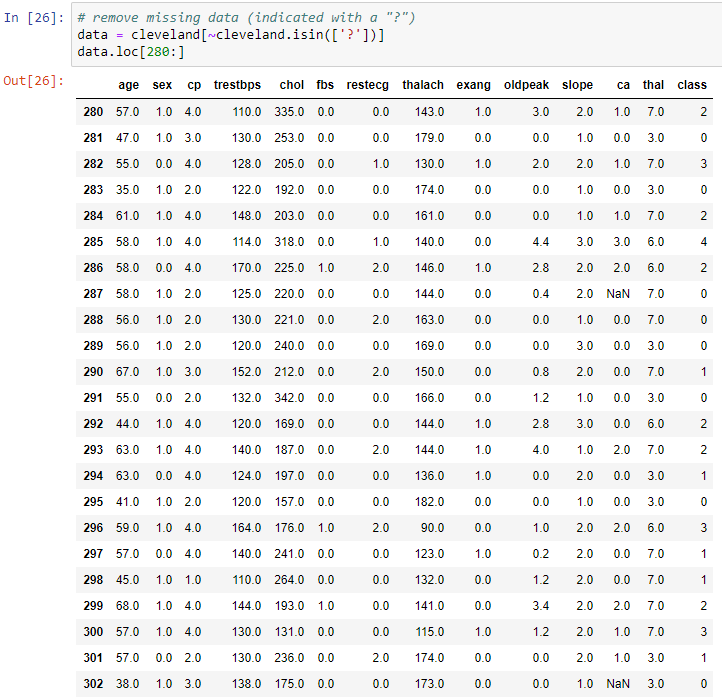
We can see, from the data in our new DataFrame, that the question marks have been replaced with NaN. We have nothing in those particular locations. Consequently, we're going to drop the rows with NaN values (or non-number values) from the DataFrame, which is really easy to do with pandas:
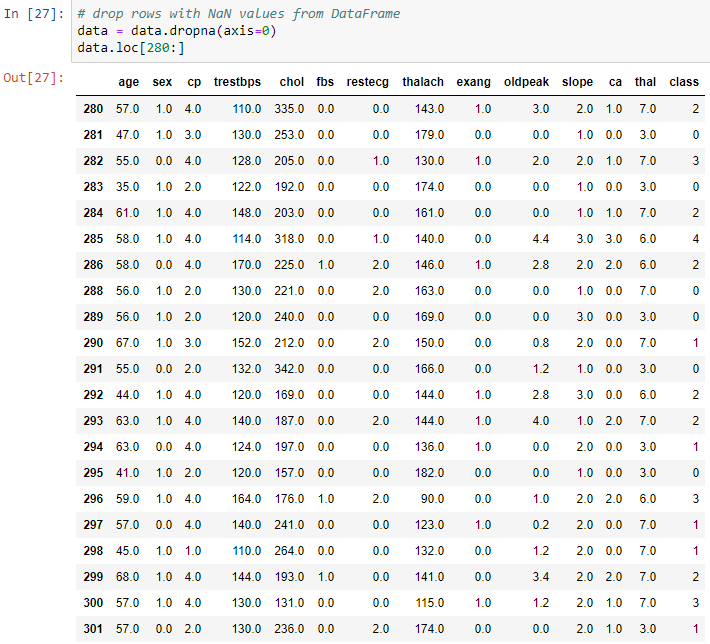
In the preceding screenshot, we use the dropna() function to drop all the missing data. As we can see, the rows...