Dilated and causal convolution
As discussed in the section on backtesting, we have to make sure that our model does not suffer from look-ahead bias:
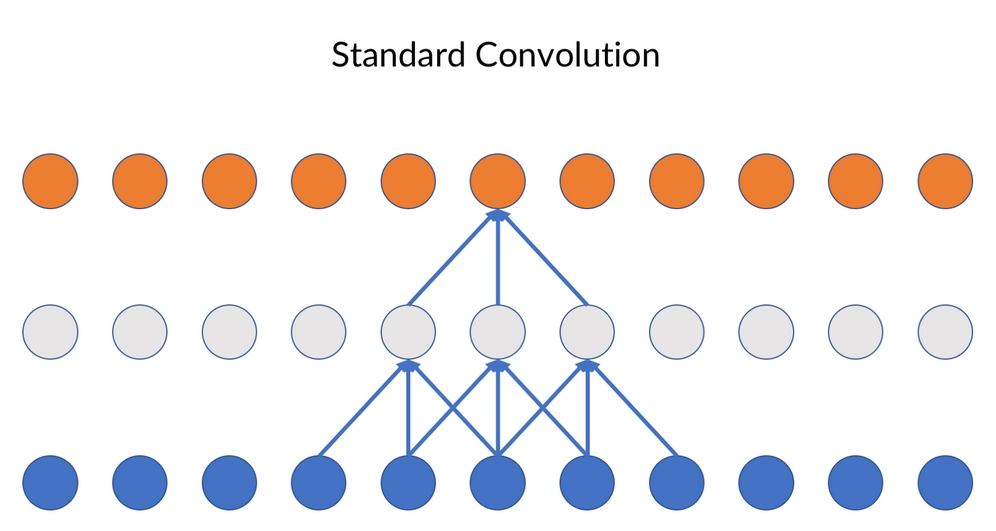
Standard convolution does not take the direction of convolution into account
As the convolutional filter slides over the data, it looks into the future as well as the past. Causal convolution ensures that the output at time t derives only from inputs from time t - 1:
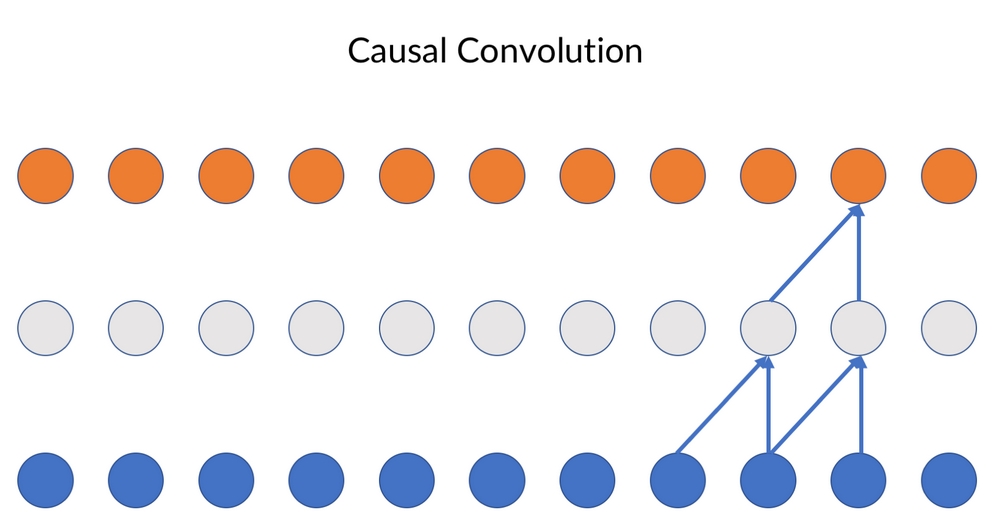
Causal convolution shifts the filter in the right direction
In Keras, all we have to do is set the padding
parameter to causal
. We can do this by executing the following code:
model.add(Conv1D(16,5, padding='causal'))
Another useful trick is dilated convolutional networks. Dilation means that the filter only accesses every nth element, as we can see in the image below.
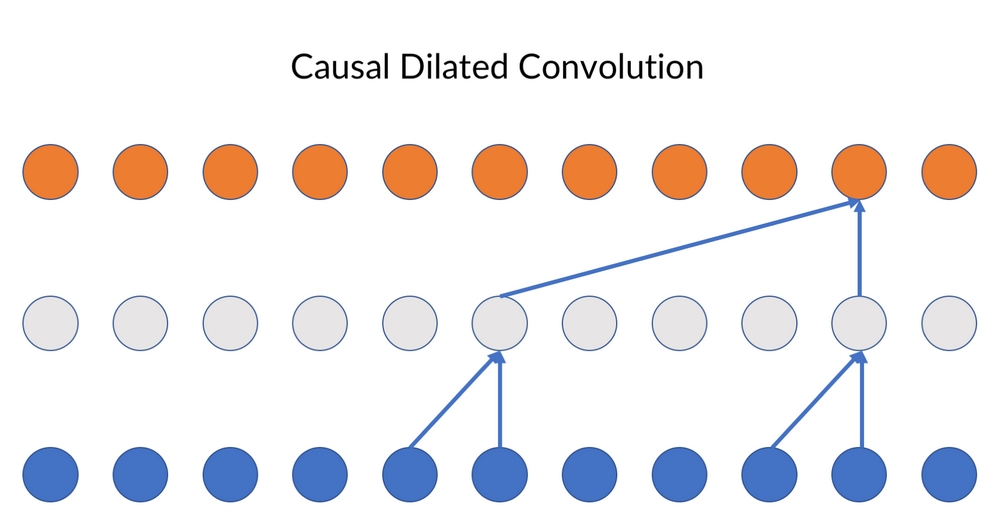
Dilated convolution skips over inputs while convolving
In the preceding diagram, the upper convolutional layer has a dilation rate of 4 and the lower layer a dilation rate of 1. We can set the dilation rate in Keras by running...