A forward pass
Over the course of this book, we will build powerful neural networks that are able to approximate extremely complex functions. We will be mapping text to named entities, images to their content, and even news articles to their summaries. But for now, we will work with a simple problem that can be solved with logistic regression, a popular technique used in both economics and finance.
We will be working with a simple problem. Given an input matrix, X, we want to output the first column of the matrix, X1. In this example, we will be approaching the problem from a mathematical perspective in order to gain some intuition for what is going on.
Later on in this chapter, we will implement what we have described in Python. We already know that we need data to train a neural network, and so the data, seen here, will be our dataset for the exercise:
X1 |
X2 |
X3 |
y |
---|---|---|---|
0 |
1 |
0 |
0 |
1 |
0 |
0 |
1 |
1 |
1 |
1 |
1 |
0 |
1 |
1 |
0 |
In the dataset, each row contains an input vector, X, and an output, y.
The data follows the formula:
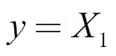
The function we want to approximate is as follows:
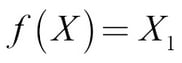
In this case, writing down the function is relatively straightforward. However, keep in mind that in most cases it is not possible to write down the function, as functions expressed by deep neural networks can become very complex.
For this simple function, a shallow neural network with only one layer will be enough. Such shallow networks are also called logistic regressors.