Weights in an ANN are the most important factor in converting an input to impact the output. This is similar to slope in linear regression, where a weight is multiplied to the input to add up to form the output. Weights are numerical parameters which determine how strongly each of the neurons affects the other.
For a typical neuron, if the inputs are x1, x2, and x3, then the synaptic weights to be applied to them are denoted as w1, w2, and w3.
Output is
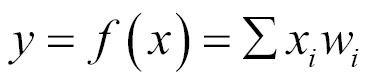
where i is 1 to the number of inputs.
Simply, this is a matrix multiplication to arrive at the weighted sum.
Bias is like the intercept added in a linear equation. It is an additional parameter which is used to adjust the output along with the weighted sum of the inputs to the neuron.
The processing done by a neuron is thus denoted as :

A function is applied on this output and is called an activation function. The input of the next layer is the output of the neurons in the previous layer, as shown in the following image:
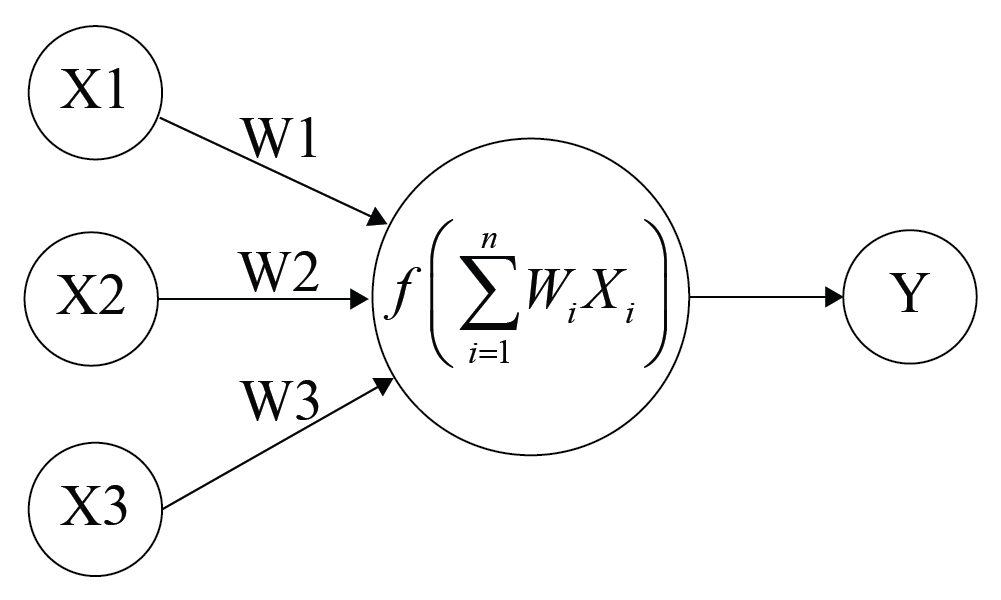