Mixture of experts
The idea behind mixture of experts is to use a set of linear regressions for each sub space of the original data space and combine them with weighting functions that will successively give weight to each linear regression.
Consider the following example dataset, which we generate with the following toy code:
x1=runif(40,0,10) x2=runif(40,10,20) e1 = rnorm(20,0,2) e2 = rnorm(20,0,3) y1 = 1+2.5*x1 + e1 y2 = 35+-1.5*x2 + e2 xx=c(x1,x2) yy=c(y1,y2)
Plotting the result, and doing a simple linear regression on it, gives the following:
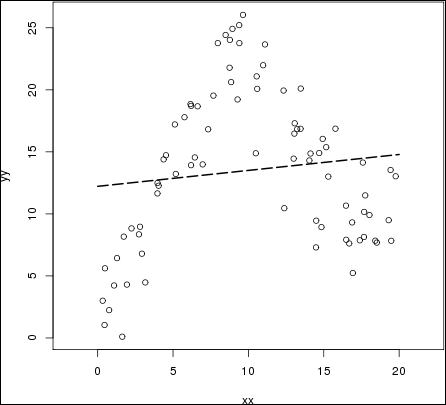
Obviously, the linear regression does not capture the behavior of the data at all. It barely captures a general trend in the data that more or less averages the data set.
The idea of mixture of experts is to have several sub models within a bigger model—for example, having several regression lines, as the following graph:
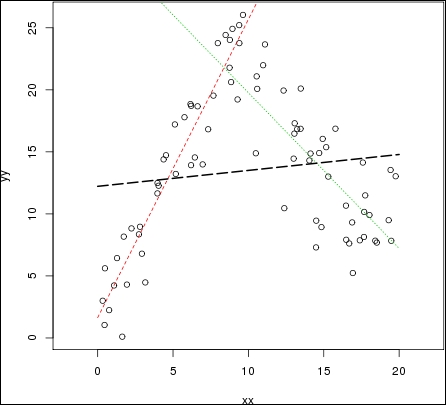
In this graph, the red and green lines seems to better represent the data set. However, the model needs to choose when to choose...