For any reinforcement learning problem, two fundamental definitions concerning the problem are important, irrespective of the learning algorithm we use. They are the definitions of the state space and the action space. We mentioned earlier in this book that the state and action spaces could be discrete or continuous. Typically, in most problems, the state space consists of continuous values and is represented as a vector, matrix, or tensor (a multi-dimensional matrix). Problems and environments with discrete action spaces are relatively easy compared to continuous valued problems and environments. In this book, we will develop learning algorithms for a few problems and environments with a mix of state space and action space combinations so that you are comfortable dealing with any such variation when you start out on your own and develop...




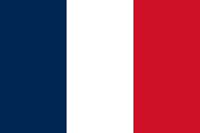


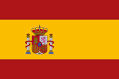



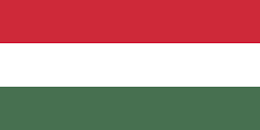

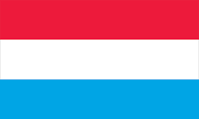





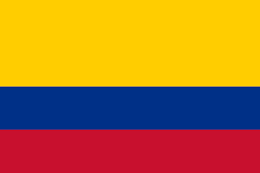
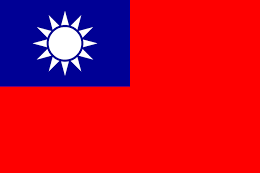
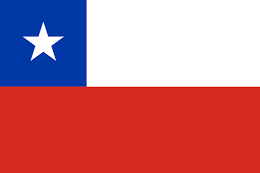



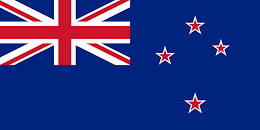
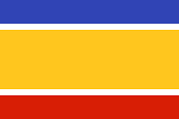
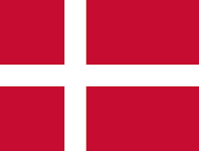
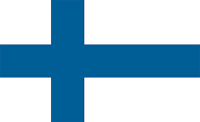







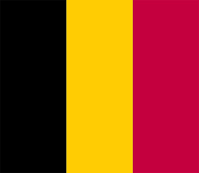

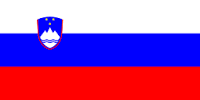





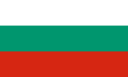


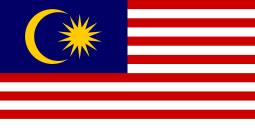



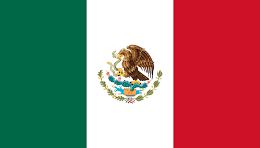
