It is common practice (as you will see next through the code examples of this chapter and from Chapter 7, Training Neural Networks with Spark, onward) to periodically insert a pooling layer between successive convolution layers in a CNN model. This kind of layers scope is to progressively reduce the number of parameters for the network (which translates into a significant lowering of the computation costs). In fact, spatial pooling (which is also found in literature as downsampling or subsampling) is a technique that reduces the dimensionality of each feature map, while at the same time retaining the most important part of the information. Different types of spatial pooling exist. The most used are max, average, sum, and L2-norm.
Let's take as an example, max pooling. This technique requires defining a spatial neighborhood (typically a 2 × 2 window);...




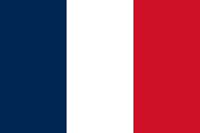


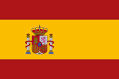



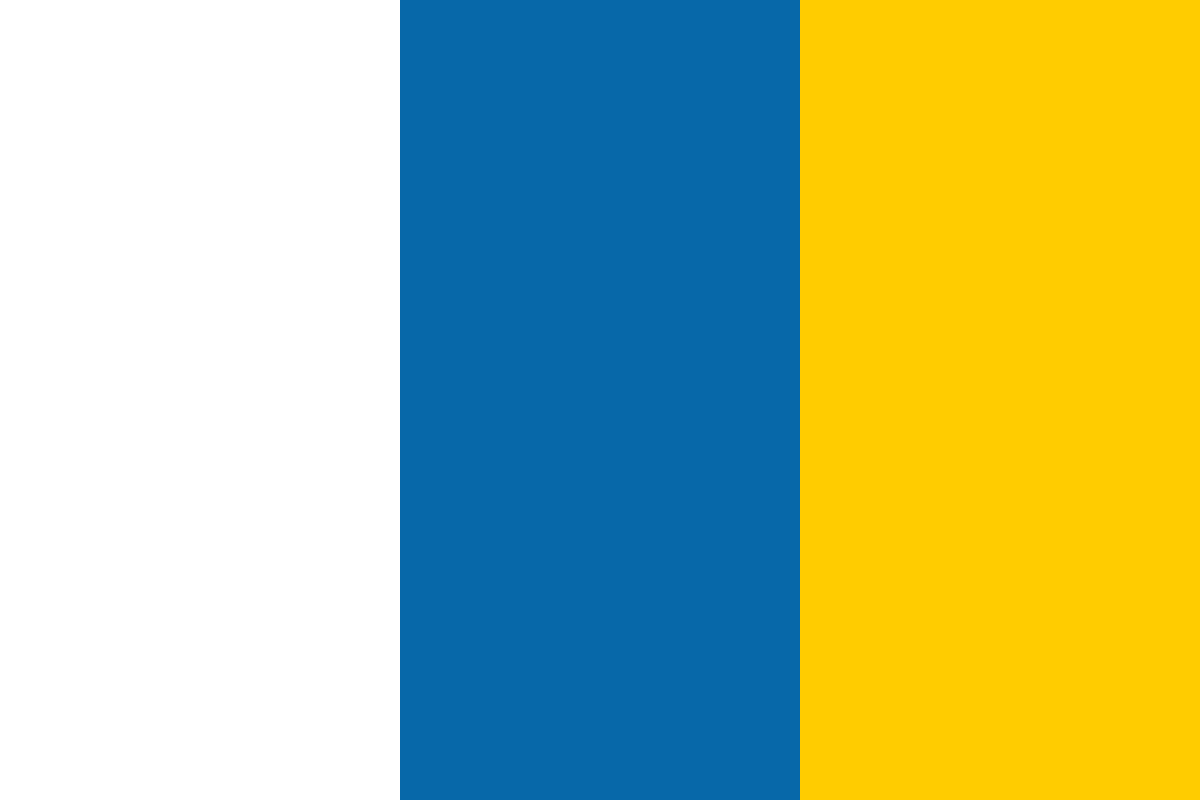
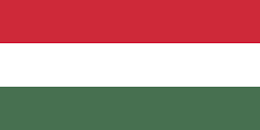

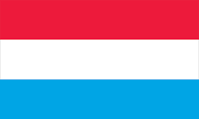





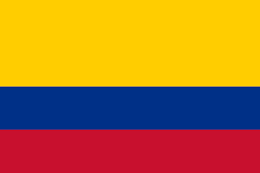
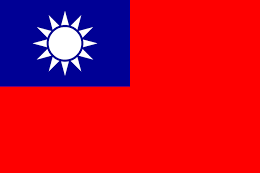
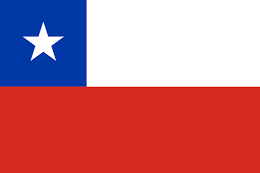



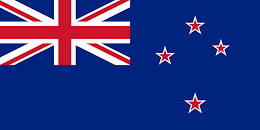
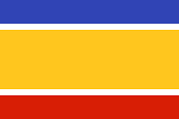
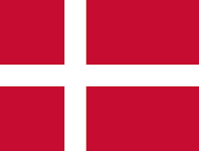
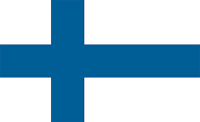







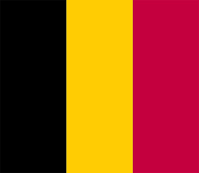

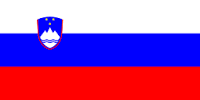





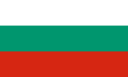


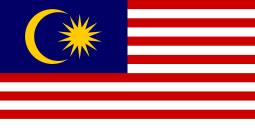



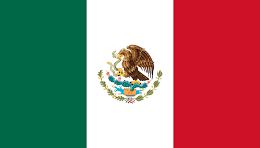
