- Unlike gradient descent, in SGD, in order to update the parameter, we don't have to iterate through all the data points in our training set. Instead, we just iterate through a single data point. That is, unlike gradient descent, we don't have to wait to update the parameter of the model after iterating all the data points in our training set. We just update the parameters of the model after iterating through every single data point in our training set.
- In mini-batch gradient descent, instead of updating the parameters after iterating each training sample, we update the parameters after iterating some batches of data points. Let's say the batch size is 50, which means that we update the parameter of the model after iterating through 50 data points, instead of updating the parameter after iterating through each individual...




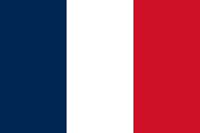


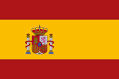



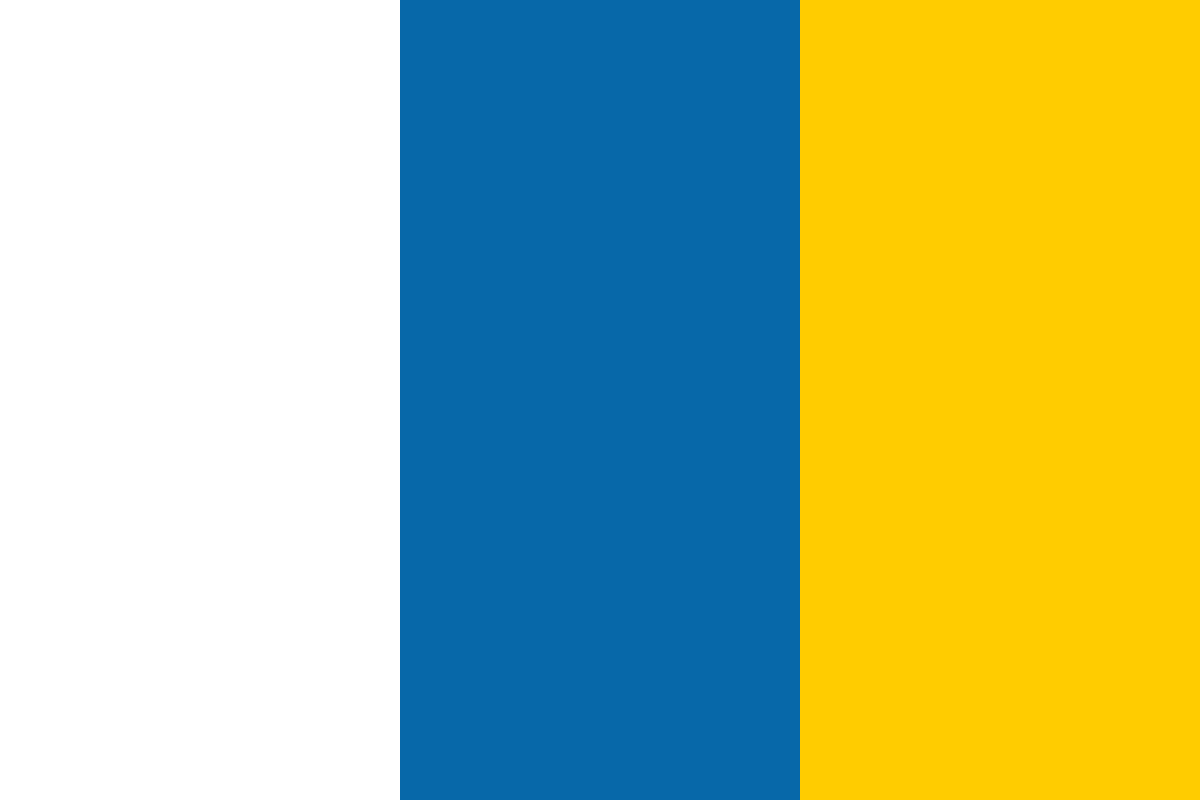
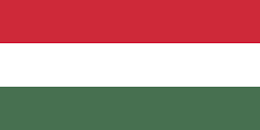

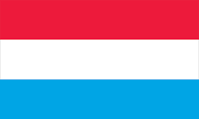





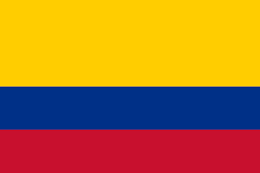
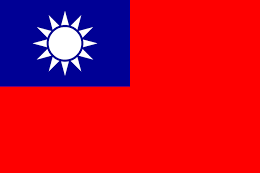
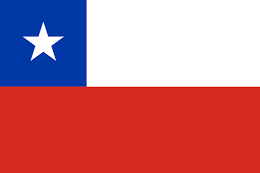



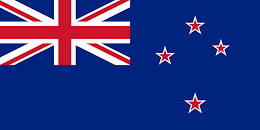
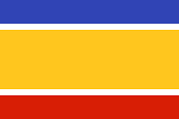
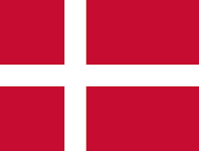
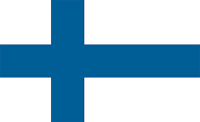







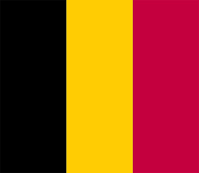

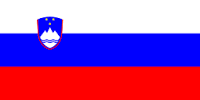





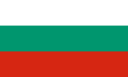


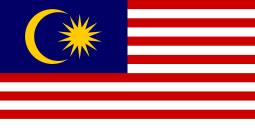



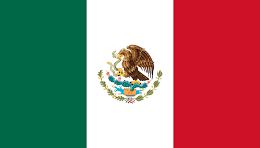
