



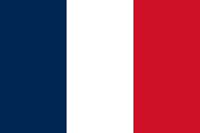


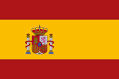



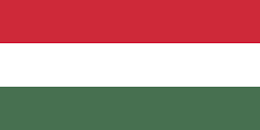

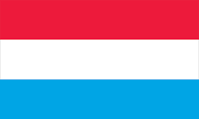





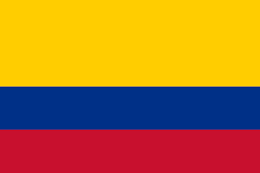
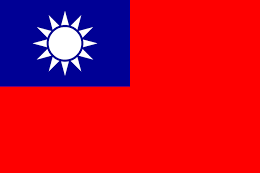
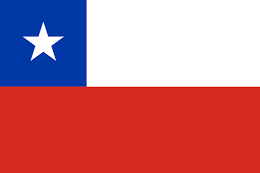



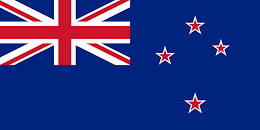
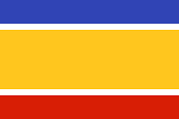
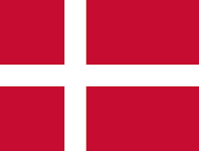
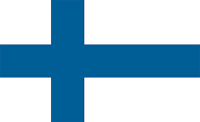







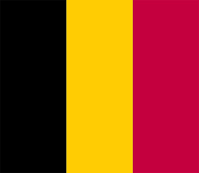

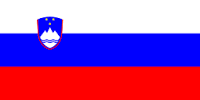





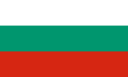


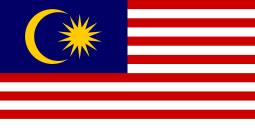



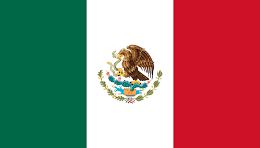

The year was 2012. Harvard Business Review had famously declared the role of data scientist as the ‘sexiest job of the 21st century’. Companies were slowly working with more data than ever before. The real actionable value of the data that could be used for commercial purposes was slowly beginning to uncover. Someone who could derive these actionable insights from the data was needed. The demand for data scientists was higher than ever.
Fast forward to 2018 - more data has been collected in the last 2 years than ever before. Data scientists are still in high demand, and the need for insights is higher than ever. There has been one significant change, though - the process of deriving insights has become more complex. If you ask the data scientists, the first initial phase of this process, which involves data cleansing, has become a lot more cumbersome. So much so, that it is no longer a myth that data scientists spend almost 80% of their time cleaning and readying the data for analysis.
In the recently conducted Packt Skill-Up survey, we asked data professionals what the worst part of the data analysis process was, and a staggering 50% responded with data cleaning.
Source: Packt Skill Up Survey
We dived deep into this, and tried to understand why many data science professionals have this common feeling of dislike towards data cleaning, or scrubbing - as many call it.
Read the Skill Up report in full. Sign up to our weekly newsletter and download the PDF for free.
Organizations these days work with a lot of data. Some of it is in a structured, readily understandable format. This kind of data is usually quite easy to clean, parse and analyze. However, some of the data is really messy, and cannot be used as is for analysis. This includes missing data, irregularly formatted data, and irrelevant data which is not worth analyzing at all.
There is also the problem of working with unstructured data which needs to be pre-processed to get the data worth analyzing. Audio or video files, email messages, presentations, xml documents and web pages are some classic examples of this.
The volume of data that businesses deal with on a day to day basis is in the scale of terabytes or even petabytes. Making sense of all this data, coming from a variety of sources and in different formats is, undoubtedly, a huge task. There are a whole host of tools designed to ease this process today, but it remains an incredibly tricky challenge to sift through the large volumes of data and prepare it for analysis.
Data cleansing can be quite an exhaustive and time-consuming task, especially for data scientists. Cleaning the data requires removal of duplications, removing or replacing missing entries, correcting misfielded values, ensuring consistent formatting and a host of other tasks which take a considerable amount of time.
Once the data is cleaned, it needs to be placed in a secure location. Also, a log of the entire process needs to be kept to ensure the right data goes through the right process. All of this requires the data scientists to create a well-designed data scrubbing framework to avoid the risk of repetition. All of this is more of a grunt work and requires a lot of manual effort. Sadly, there are no tools in the market which can effectively automate this process.
Given that data cleaning is a rather tedious job, many businesses think of outsourcing the task to third party vendors. While this reduces a lot of time and effort on the company’s end, it definitely increases the cost of the overall process. Many small and medium scale businesses may not be able to afford this, and thus are heavily reliant on the data scientist to do the job for them.
It is quite obvious that data scientists need clean, ready-to-analyze data if they are to to extract actionable business insights from it. Some data scientists equate data cleaning to donkey work, suggesting there’s not a lot of innovation involved in this process. However, some believe data cleaning is rather important, and pay special attention to it given once it is done right, most of the problems in data analysis are solved.
It is very difficult to take advantage of the intrinsic value offered by the dataset if it does not adhere to the quality standards set by the business, making data cleaning a crucial component of the data analysis process.
Now that you know why data cleaning is essential, why not dive deeper into the technicalities? Check out our book Practical Data Wrangling for expert tips on turning your noisy data into relevant, insight-ready information using R and Python.
Cleaning Data in PDF Files
30 common data science terms explained
How to create a strong data science project portfolio that lands you a job