In this section, we will extend decision trees to random forests, which are an example of an approach to machine learning called ensemble learning. We also see how we can train these models when applying them to the Titanic dataset.
In ensemble learning, we train multiple classifiers for our dataset. For random forests, we train decision trees on random subsets of the dataset. The classifier is fed a data point that we want to predict the class for. The data point is fed to each classifier that's trained on the dataset. Each classifier then makes a prediction. The predictions are then aggregated in some way to form a final prediction. This diagram shows the steps to do this:
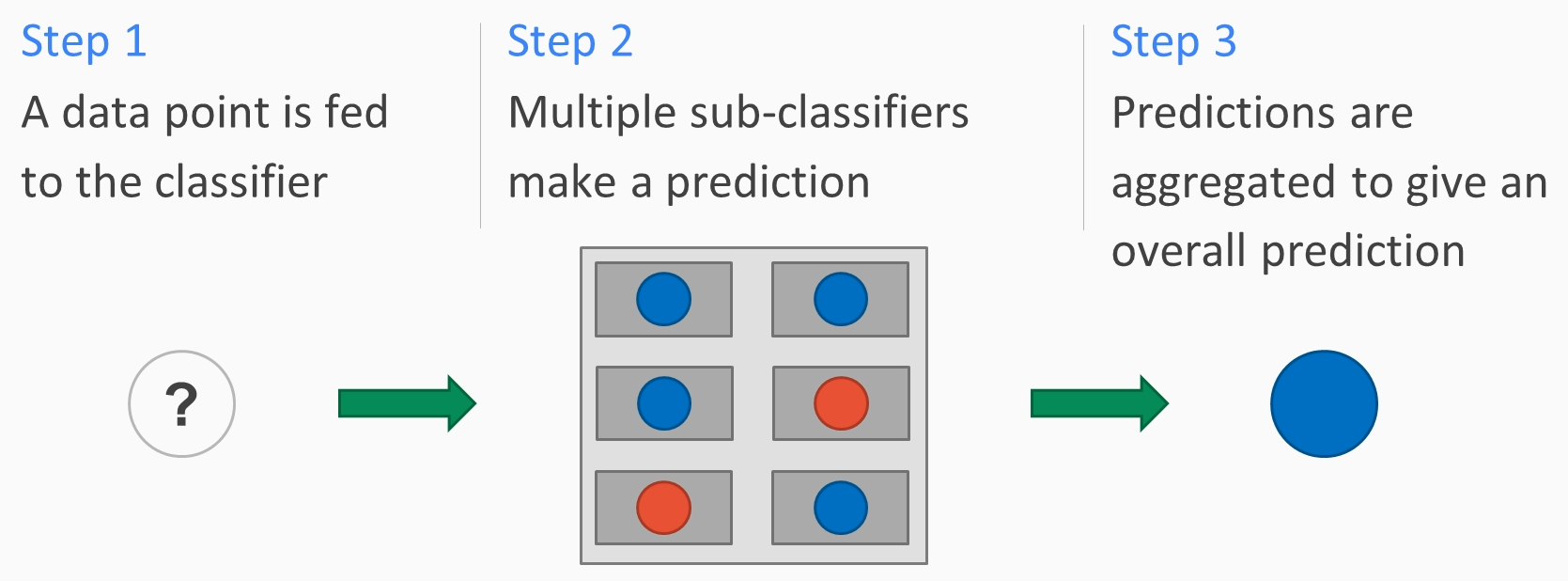
For random forests, the class that was predicted the most often is the final prediction. Let's get right into the action by following these steps:
- First, let's load in the Titanic...