Understanding SVMs
Without going into details of how an SVM works, let's just try to grasp what it can help us accomplish in the context of machine learning and computer vision. Given labeled training data, an SVM learns to classify the same kind of data by finding an optimal hyperplane, which, in plain English, is the plane that divides differently labeled data by the largest possible margin. To aid our understanding, let's consider the following diagram, which is provided by Zach Weinberg under the Creative Commons Attribution-Share Alike 3.0 Unported License:
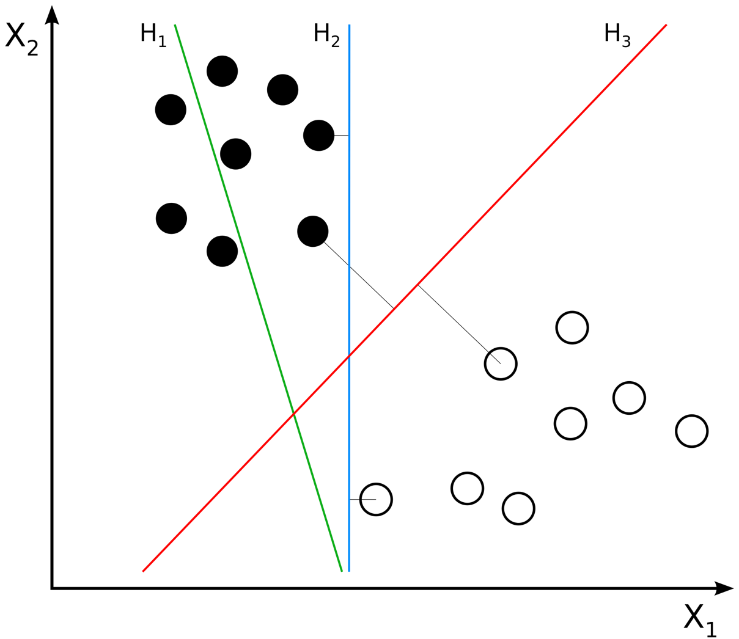
Hyperplane H1 (shown as a green line) does not divide the two classes (the black dots versus the white dots). Hyperplanes H2 (shown as a blue line) and H3 (shown as a red line) both divide the classes; however, only hyperplane H3 divides the classes by a maximal margin.
Let's suppose we are training an SVM as a people detector...