- The true probability distribution of an event is the actual likelihood of encountering that event. We discover this distribution through repeated experimental trials.
- Conducting more trials gives us a better picture of the true probability distribution of a problem. In most problem spaces, conducting 10 trials of an event would not give us sufficient data to develop a detailed model of the event.
- A small sample size might be biased in a way the experimenter is not aware of, and the descriptive statistics of that sample might not be reflected in a larger sample.
- Thompson sampling is a Bayesian method for optimization that involves choosing a prior probability distribution for an event and updating that as more information about the event is received. Since it is computationally expensive to try to find the...




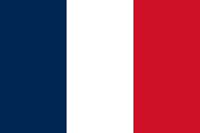


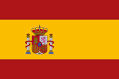



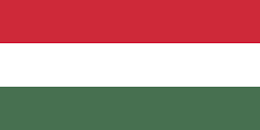

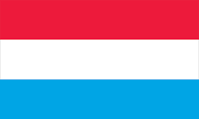





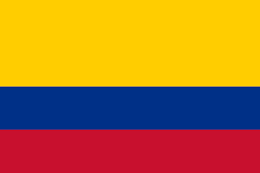
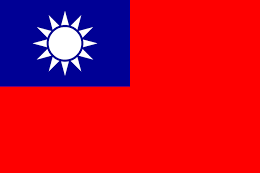
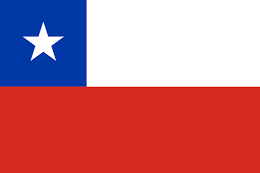



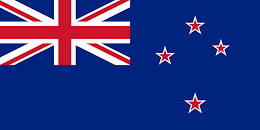
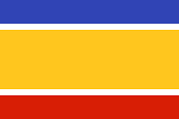
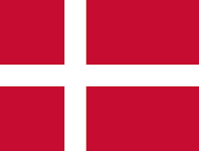
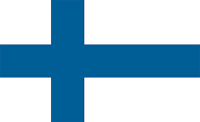







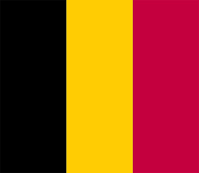

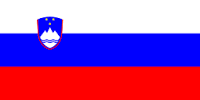





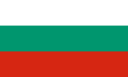


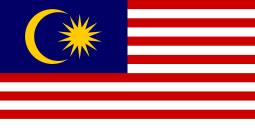



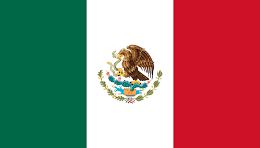
