In Chapter 1, Thinking Probabilistically, we introduced the concept of posterior predictive checks, and, in subsequent chapters, we have used it as a way to evaluate how well models explain the same data that's used to fit the model. The purpose of posterior predictive checks is not to dictate that a model is wrong; we already know that! By performing posterior predictive checks, we hope to get a better grasp of the limitations of a model, either to properly acknowledge them, or to attempt to improve the model. Implicit, in the previous statement is the fact that models will not generally reproduce all aspects of a problem equally well. This is not generally a problem given that models are built with a purpose in mind. A posterior predictive check is a way to evaluate a model in the context of that purpose; thus, if we have more than one model...




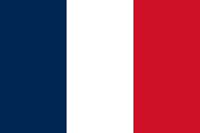


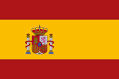



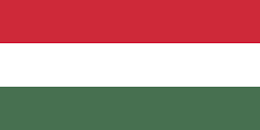

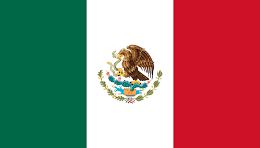


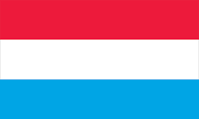



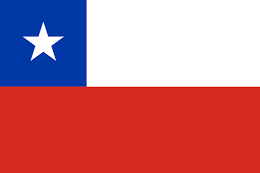


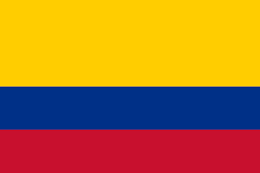
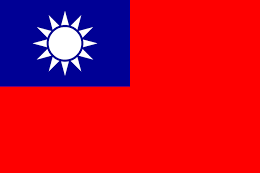


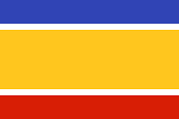
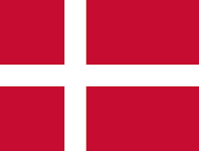
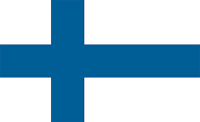



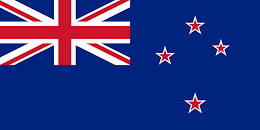





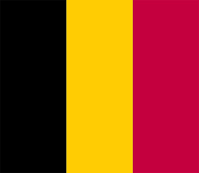

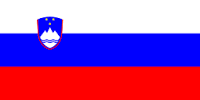




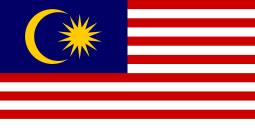


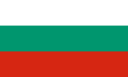


