Holdout Approach/Validation
This is the easiest approach (though not the most recommended) used in validating model performance. We have used this approach throughout the book to test our model performance in the previous chapters. Here, we randomly divide the available dataset into training and testing datasets. Most common split ratios used between the train and test datasets are 70:30 or 80:20.
The major drawbacks of this approach are that the model performance is purely evaluated from a fractional test dataset, and it might not be the best representation for the model performance. The evaluation of the model will completely depend on the type of split, and therefore, the nature of the data points that end up in the training and testing datasets, which might then lead to significantly different results and thus high variance.
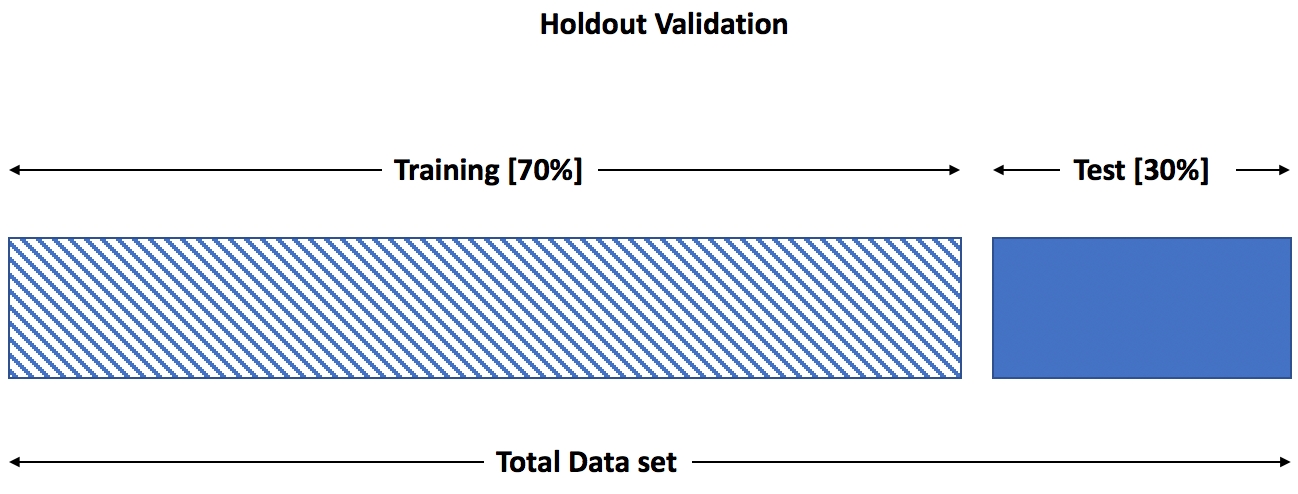
Figure 7.3: Holdout validation
The following exercise divides the dataset into 70% training and 30% testing, and builds a random forest model on the training dataset...