



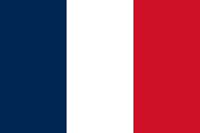


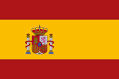



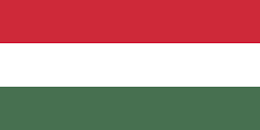

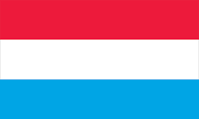





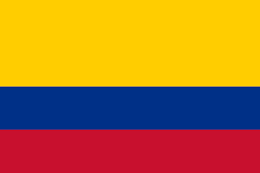
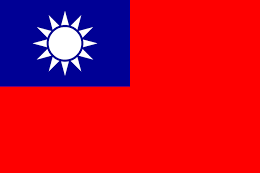
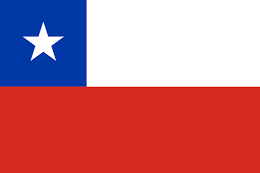



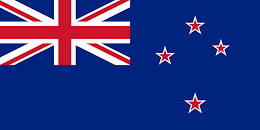
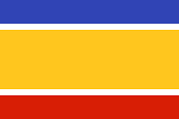
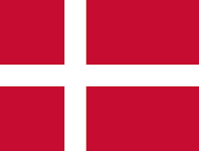
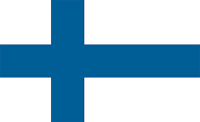







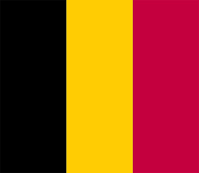

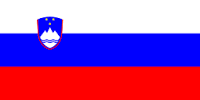





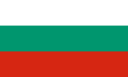


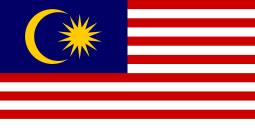



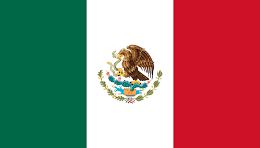

One of the prominent minds in AI, Andrew Ng believes Factories to be AI's next frontier! Andrew is on a mission to AI-ify manufacturing with its new start-up called Landing.AI. For this initiative he partnered with Foxconn, world's largest contract manufacturer and makers of Apple iPhones. Together they aim to develop a wide range of AI transformation programs, from introduction of new technologies, operational processes, automated quality control and much more.
In this AI-powered industrial revolution, machines are becoming smarter and interconnected. Manufacturers are using embedded intelligence of machines for collecting and analyzing data to generate meaningful insights. These are then used to run equipment efficiently, optimize workflows of operations and supply chains, among other things. Thus, AI is leaving an indelible impact across the manufacturing cycle. Further, a new wave of automation is transforming the role of human workforce wherein AI-driven robots are empowering production 24-hours a day. This is helping industrial environments gear up for the shift towards the smart factory environment. Below are some ways AI is revolutionizing manufacturing.
Smart manufacturing systems are leveraging the power of predictive analysis and machine learning algorithms to enhance the production capacity. Predictive analytics derives its power from the data collected from the devices or sensors embedded in a manufacturer’s industrial equipments. These sensors become part of the IoT (Internet of Things) which collects and shares data with data scientists on the cloud.
This setup is helping manufacturing industries to move from repair-and-replace to a predict-and-fix maintenance model. They do this by enabling these businesses to retrieve the right information at the right time to make the right decisions. For instance, in a pump manufacturing company, data scientists could collect, store and analyze sensor data based on machine attributes like heat, vibration, noise etc. This data can be stored in the cloud allowing for an array of analyses to be performed from understanding machine performance to predicting and monitoring disruption in processes and equipment remotely. Further, syncing up production schedules with parts availability can ensure enhanced production output.
Manufacturers can deploy supervised/unsupervised ML, DL and reinforcement learning algorithms to monitor quality issues in the manufacturing process. For instance, researchers at Lappeenranta University of Technology in Finland have developed an innovative welding system for high-strength steel. They used unsupervised learning to allow the system to learn to mimic human’s ability to self-explore and self-correct. This welding system detects imperfections and self-corrects during the welding process using a new kind of sensor system controlled by a neural network program. Further, it also calculates other faults that may arise during the entire process.
Visual inspection technology in an industrial environment identifies both functional and cosmetic defects. IBM has developed a new offering for manufacturing clients to automate visual quality inspections. Rooted in deep learning, a centralized ‘learning service’ collects images of all products - normal and abnormal. Next, it builds analytical models to recognize and classify different characteristics of machine parts and components into OK or NG. Characteristics that meet quality specifications are considered as OK while those that don't are classified as ‘NG’.
Manufacturing industries strive to achieve excellence throughout the production process. To ensure this, machinery embedded with sensors generate real-time performance and workload data. This helps in diagnosing faults and in predicting the need for equipment maintenance. For instance, a machine may break down due to lack of maintenance in the long run, incurring losses to business. With predictive maintenance, businesses can be better equipped to handle equipment malfunction by identifying significant causal factors like weather, temperature etc.
Targeted predictive maintenance generates critical information such as which machine parts will need replacing and when. This helps in reducing equipment downtime, lowering maintenance costs and pre-emptively addressing aging equipment.
Large warehouses face challenging times in streamlining space, managing inventories and reducing transit time. Manufacturing industries are employing reinforcement learning for efficient warehouse management. RL approach uses trial and error iterations within an environment to achieve a particular goal.
Imagine what a breeze warehousing could be and the associated cost savings, if robots could pick up the right products from various lots and move them to the right destinations with great precision. Here, reinforcement learning based algorithms can improve the efficiency of such intelligent warehouses with multirobot systems by addressing task scheduling and path planning issues. Fanuc, a Tokyo based company, employs robots having reinforced learning ability to perform such tasks with great agility and precision.
AI is helping manufacturers gain an in-depth understanding of the complex variables at play in the supply chain and in predicting future scenarios. To enable seamless insights generation, businesses are opting for more flexible and efficient cyber-physical systems. These intelligent systems are self-configurative and self-optimizing structures that can predict problems and minimize losses. Thus they help businesses to innovate rapidly by reducing the time to market, foresee uncertainties and deal with them promptly. Siemens, for example, is creating a self-organizing factory that aims to automate the entire supply chain by generating work orders using the demand and order information.
Industry 4.0 is the new way of manufacturing using automation, devices connected on the IoT, cloud and cognitive computing. It propagates the concept of the “smart factory” in which cyber-physical systems observe the physical processes of the factory and make discrete decisions accordingly.
As AI finds its application in Industry 4.0, computers will merge together with robotics to automate and maximize the efficiency of the industrial processes. Powered by machine learning algorithms, the computer systems could control the robots with minimum human intervention. For instance, in a manufacturing setup, AI can work alongside systems like SCADA - to control industrial processes in an efficient manner. These systems can monitor, collect and process real-time data by directly interacting with devices such as sensors, pumps, motors etc. through human-machine interface (HMI) software. These machine-to-machine communication systems give new direction to the human-machine collaboration potential thus changing the way we see workforce management. Industry 4.0 will favor those who can build software, hardware, and firmware - those who can adapt and maintain new equipment and those who can design automation and robotics.
Within Industry 4.0, augmented reality and virtual reality are other cutting edge production ready technologies that are making the idea of a smart factory a reality. The recent relaunch of Google Glass especially designed for the factory floor is worth a mention here. The Wi-Fi-enabled glasses allow factory workers, mechanics, and other technicians to view instructional videos, manuals, training videos etc., all in their line of sight. This helps in maintaining higher standards of work while ensuring safety with agility.
Manufacturing industries are gearing themselves to harness AI along with IoT, AR/VR to create an agile manufacturing environment and to make smarter and real-time decisions. AI is helping realize the full potential of Industrial Internet of Things (IIoT) by applying machine learning, deep learning and other evolutionary algorithms to the sensor data. Human-machine collaboration is transforming the scenario at the fulfillment centers creating a win-win situation for both humans and robots. Robots employed at the fulfillment centers having motion sensors move on to the field of QR codes with precision and agility withouting running into each other creating a fascinating view.
Imagine a real-life JARVIS from the movie Iron Man managing entire supply chains or factory spaces. The day is not far away when we can see a JARVIS like advanced virtual assistant uses sensors to collect real-time data, AI to process data,and blockchain to securely transmit the information while using AR to interact with us visually. It could take care of system and mechanical failures remotely while ceasing control of the factory for efficient energy management. Manufacturers could go save the world or unveil new products, Iron Man style!