Implementing an autoencoder
For autoencoders, we use a network architecture, as shown in the following figure. In the first couple of layers, we decrease the number of hidden units. Halfway, we start increasing the number of hidden units again until the number of hidden units is the same as the number of input variables. The middle hidden layer can be seen as an encoded variant of the inputs, where the output determines the quality of the encoded variant:
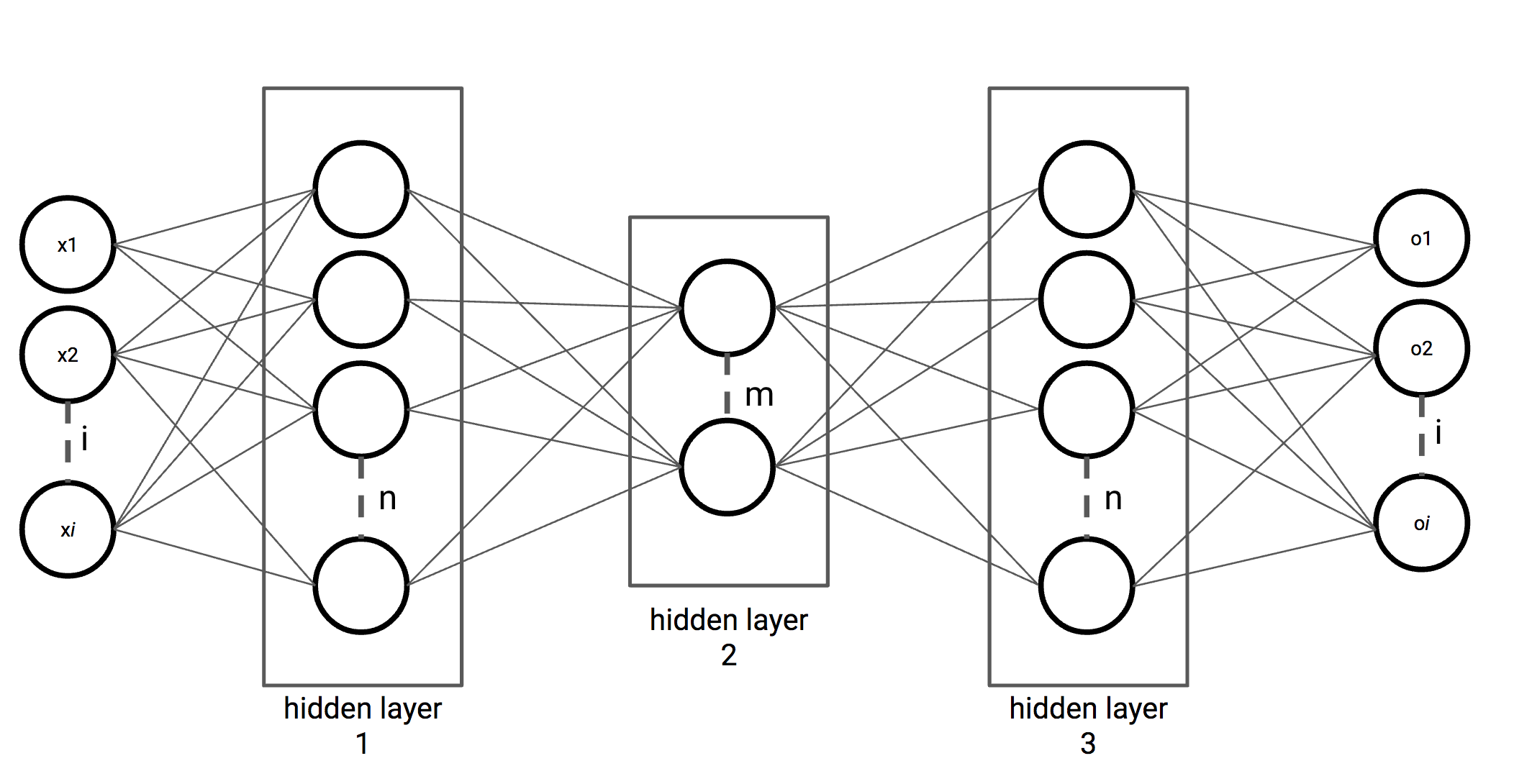
Figure 2.13: Autoencoder network with three hidden layers, with m < n
In the next recipe, we will implement an in Keras to decode Street View House Numbers (SVHN) from 32 x 32 images to 32 floating numbers. We can determine the quality of the encoder by decoding back to 32 x 32 and comparing the images.
How to do it...
- Import the necessary libraries with the following code:
import numpy as np from matplotlib import pyplot as plt import scipy.io from keras.models import Sequential from keras.layers.core import Dense from keras.optimizers...