Chapter 1. How to Sound Like a Data Scientist
No matter which industry you work in—IT, fashion, food, or finance—there is no doubt that data affects your life and work. At some point this week, you will either have or hear a conversation about data. News outlets are covering more and more stories about data leaks, cybercrimes, and how data can give us a glimpse into our lives. But why now? What makes this era such a hotbed of data-related industries?
In the nineteenth century, the world was in the grip of the Industrial Age. Mankind was exploring its place in the industrial world, working with giant mechanical inventions. Captains of industry, such as Henry Ford, recognized that using these machines could open major market opportunities, enabling industries to achieve previously unimaginable profits. Of course, the Industrial Age had its pros and cons. While mass production placed goods in the hands of more consumers, our battle with pollution also began at around this time.
By the twentieth century, we were quite skilled at making huge machines; the goal now was to make them smaller and faster. The Industrial Age was over and was replaced by what we now refer to as the Information Age. We started using machines to gather and store information (data) about ourselves and our environment for the purpose of understanding our universe.
Beginning in the 1940s, machines such as ENIAC (considered one of the first—if not the first—computers) were computing math equations and running models and simulations like never before. The following photograph shows ENIAC:
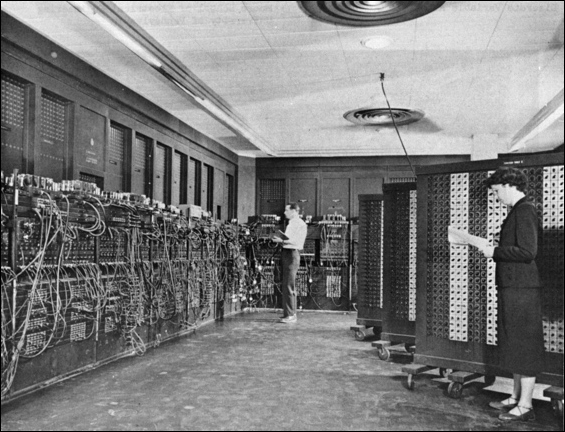
ENIAC—The world's first electronic digital computer (Ref: http://ftp.arl.mil/ftp/historic-computers/)
We finally had a decent lab assistant who could run the numbers better than we could! As with the Industrial Age, the Information Age brought us both the good and the bad. The good was the extraordinary works of technology, including mobile phones and televisions. The bad was not as bad as worldwide pollution, but still left us with a problem in the twenty-first century—so much data.
That's right—the Information Age, in its quest to procure data, has exploded the production of electronic data. Estimates show that we created about 1.8 trillion gigabytes of data in 2011 (take a moment to just think about how much that is). Just one year later, in 2012, we created over 2.8 trillion gigabytes of data! This number is only going to explode further to hit an estimated 40 trillion gigabytes of created data in just one year by 2020. People contribute to this every time they tweet, post on Facebook, save a new resume on Microsoft Word, or just send their mom a picture by text message.
Not only are we creating data at an unprecedented rate, but we are also consuming it at an accelerated pace as well. Just five years ago, in 2013, the average cell phone user used under 1 GB of data a month. Today, that number is estimated to be well over 2 GB a month. We aren't just looking for the next personality quiz—what we are looking for is insight. With all of this data out there, some of it has to be useful to me! And it can be!
So we, in the twenty-first century, are left with a problem. We have so much data and we keep making more. We have built insanely tiny machines that collect data 24/7, and it's our job to make sense of it all. Enter the Data Age. This is the age when we take machines dreamed up by our nineteenth century ancestors and the data created by our twentieth century counterparts and create insights and sources of knowledge that every human on Earth can benefit from. The United States created an entirely new role in the government of chief data scientist. Many companies are now investing in data science departments and hiring data scientists. The benefit is quite obvious—using data to make accurate predictions and simulations gives us insight into our world like never before.
Sounds great, but what's the catch?
This chapter will explore the terminology and vocabulary of the modern data scientist. We will learn keywords and phrases that will be essential in our discussion of data science throughout this book. We will also learn why we use data science and learn about the three key domains that data science is derived from before we begin to look at the code in Python, the primary language used in this book. This chapter will cover the following topics:
- The basic terminology of data science
- The three domains of data science
- The basic Python syntax