In this chapter, we looked specifically at one of the more state-of-the-art advances in DRL from DeepMind called Rainbow DQN. Rainbow combines several improvements layered on top of DQN that allow dramatic increases in training performance. As we have already covered many of these improvements, we only needed to review a couple of new advances. Before doing that though, we installed TensorBoard as a tool to investigate training performance. Then, we looked at the first advancement in distributional RL and how to model the action by understanding the sampling distribution. Continuing with distributions, we then looked at noisy network layers—network layers that don't have individual weights but rather individual distributions to describe each weight. Building on this example, we moved onto Rainbow DQN with our last example, finishing off with a quick discussion...




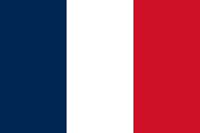


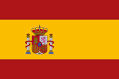



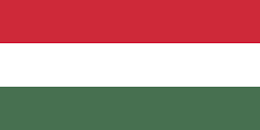

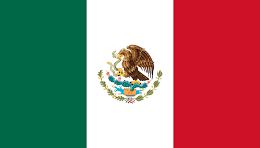


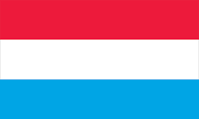



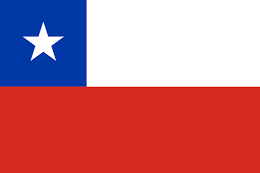


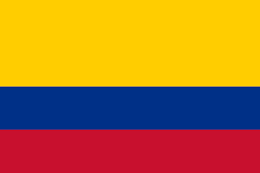
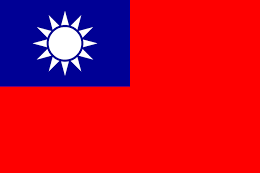


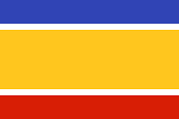
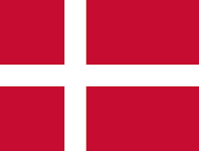
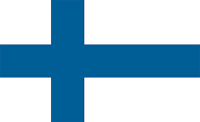



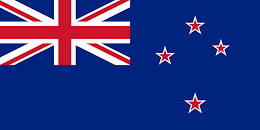





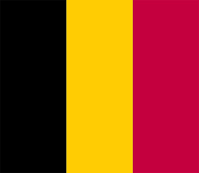

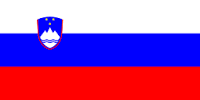




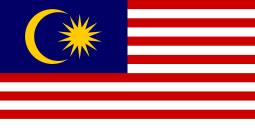


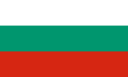


