The point of splitting the dataset into training and testing sets was to simulate the situation of using the model to make predictions on data the model has not seen. As we said before, the whole point is to generalize what we have learned from the observed data. The training MSE (or any metric calculated on the training dataset) may give us a biased view of the performance of our model, especially because of the possibility of overfitting. The metrics of performance we get from the training dataset will tend to be too optimistic. Let's take a look again at our illustration of overfitting:
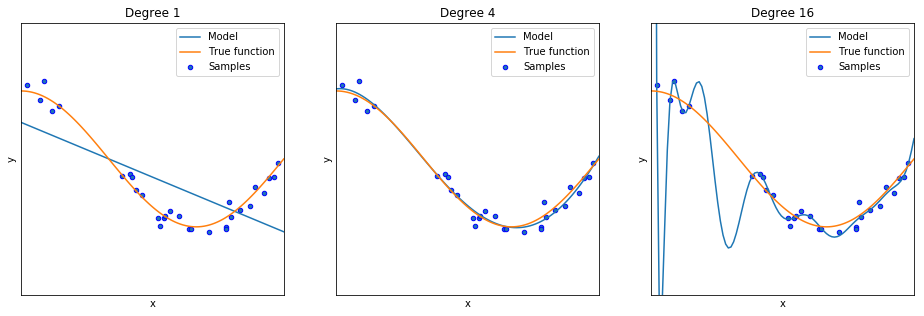
If we calculate the training MSE for these three cases, we will definitely get the lowest one (hence the best) for the third model, the polynomial with 16 degrees; as we see, the model touches many points, making the error for those points exactly 0. However...