The shift to prompt-based approaches
As discussed in prior chapters, the development of the original GPT marked a significant advance in natural language generation, introducing the use of prompts to instruct the model. This method allowed models such as GPT to perform tasks such as translations – converting text such as “Hello, how are you?” to “Bonjour, comment ça va?” – without task-specific training, leveraging deeply contextualized semantic patterns learned during pretraining. This concept of interacting with language models via natural language prompts was significantly expanded with OpenAI’s GPT-3 in 2020. Unlike its predecessors, GPT-3 showcased remarkable capabilities in understanding and responding to prompts in zero- and few-shot learning scenarios, a stark contrast to earlier models that weren’t as adept at such direct interactions. The methodologies, including the specific training strategies and datasets used for achieving GPT-3’s advanced performance, remain largely undisclosed. Nonetheless, it is inferred from OpenAI’s public research that the model learned to follow instructions based on its vast training corpus, and not explicit instruction-tuning. GPT-3’s success in performing tasks based on simple and direct prompting highlighted the potential for language models to understand and execute a wide range of tasks without requiring explicit task-specific training data for each new task. This led to a new paradigm in NLP research and applications, focusing on how effectively a model could be prompted with instructions to perform tasks such as summarization, translation, content generation, and more.
After the release of GPT-3, OpenAI was among the first to introduce specialized fine-tuning to respond more accurately to instructions in their release of InstructGPT (Ouyang et al., 2022). The researchers aimed to teach the model to closely follow instructions using two novel approaches. The first was Supervised Fine-Tuning (SFT), which involved fine-tuning using datasets carefully crafted from prompts and response pairs. These demonstration datasets were then used to perform SFT on top of the GPT-3 pretrained model, refining it to provide responses more closely aligned with human responses. Figure 7.1 provides an example of a prompt and response pair.
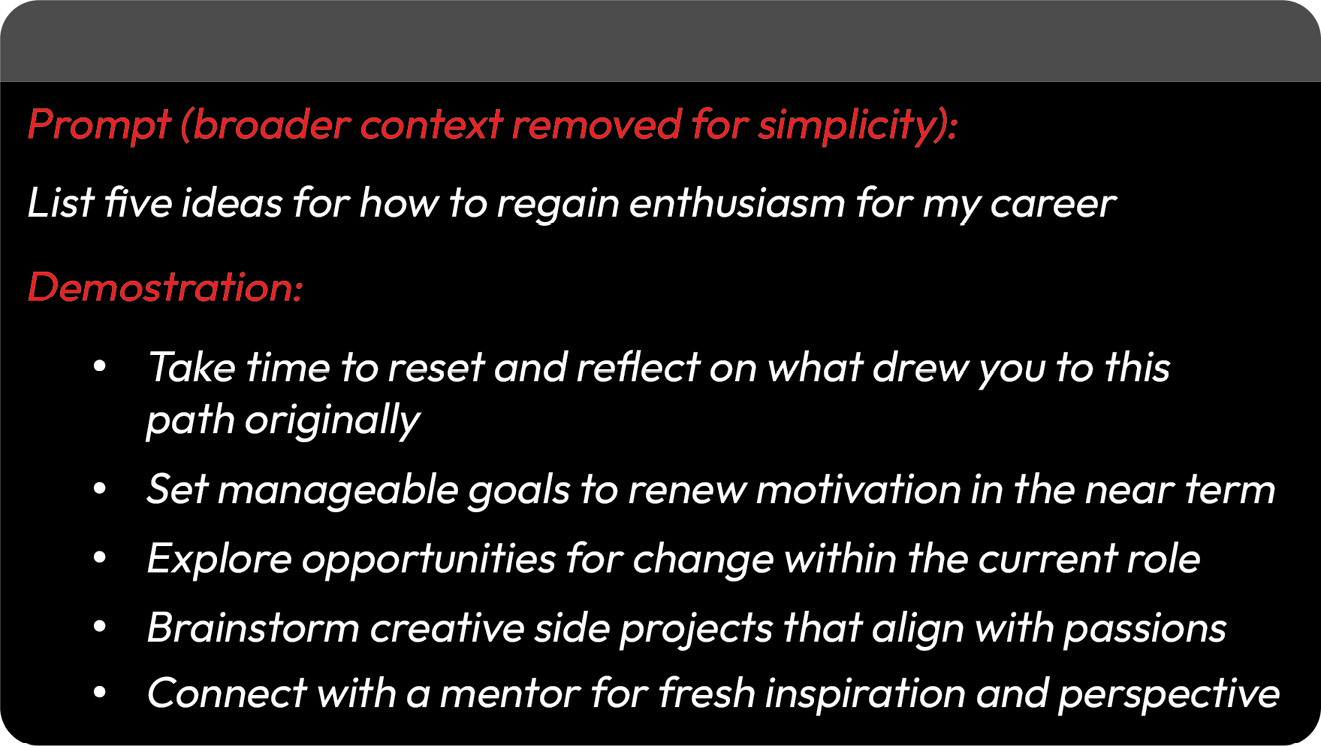
Figure 7.1: InstructGPT SFT instruction and output pairs
The second approach involved additional refinement using Reinforcement Learning from Human Feedback (RLHF). Reinforcement Learning (RL), established decades ago, aims to enhance autonomous agents’ decision-making capabilities. It does this by teaching them to optimize their actions based on the trade-off between risk and reward. The policy captures the guidelines for the agent’s behavior, dynamically updating as new insights and feedback are learned to refine decisions further. RL is the exact technology used in many robotic applications and is most famously applied to autonomous driving.
RLHF is a variation of traditional RL, incorporating human feedback alongside the usual risk/reward signals to direct LLM behavior toward better alignment with human judgment. In practice, human labelers would provide preference ratings on model outputs from various prompts, and these ratings would be used to update the model policy, steering the LLM to generate responses that better conform to expected user intent across a range of tasks. In effect, this technique helped to reduce the model’s tendency to generate inappropriate, biased, harmful, or otherwise undesirable content. Although RLHF is not a perfect solution in this regard, it represents a significant step toward models that better understand and align with human values.
Later that year, following OpenAI’s introduction of InstructGPT, Google unveiled Fine-tuned Language Net or FLAN (Wei et al., 2021). FLAN represented another leap toward prompt-based LLMs, employing explicit instruction tuning. Google’s approach relied on formatting existing datasets into instructions, enabling the model to understand various tasks. Specifically, the authors of FLAN merged multiple NLP datasets across different categories, such as translation and question answering, creating distinct instruction templates for each dataset to frame them as instruction-following tasks. For example, the FLAN team leveraged ANLI challenges (Nie et al., 2020) to construct question-answer pairs explicitly designed to test the model’s understanding of complex textual relationships and reasoning. By framing these challenges as question-answer pairs, the FLAN team could directly measure a model’s proficiency in deducing these relationships under a unified instruction-following framework. Through this innovative approach, FLAN effectively broadened the scope of tasks a model can learn from, enhancing its overall performance and adaptability across a diverse set of NLU benchmarks. Figure 7.2 presents a theoretical example of question-answer pairs based on ANLI.
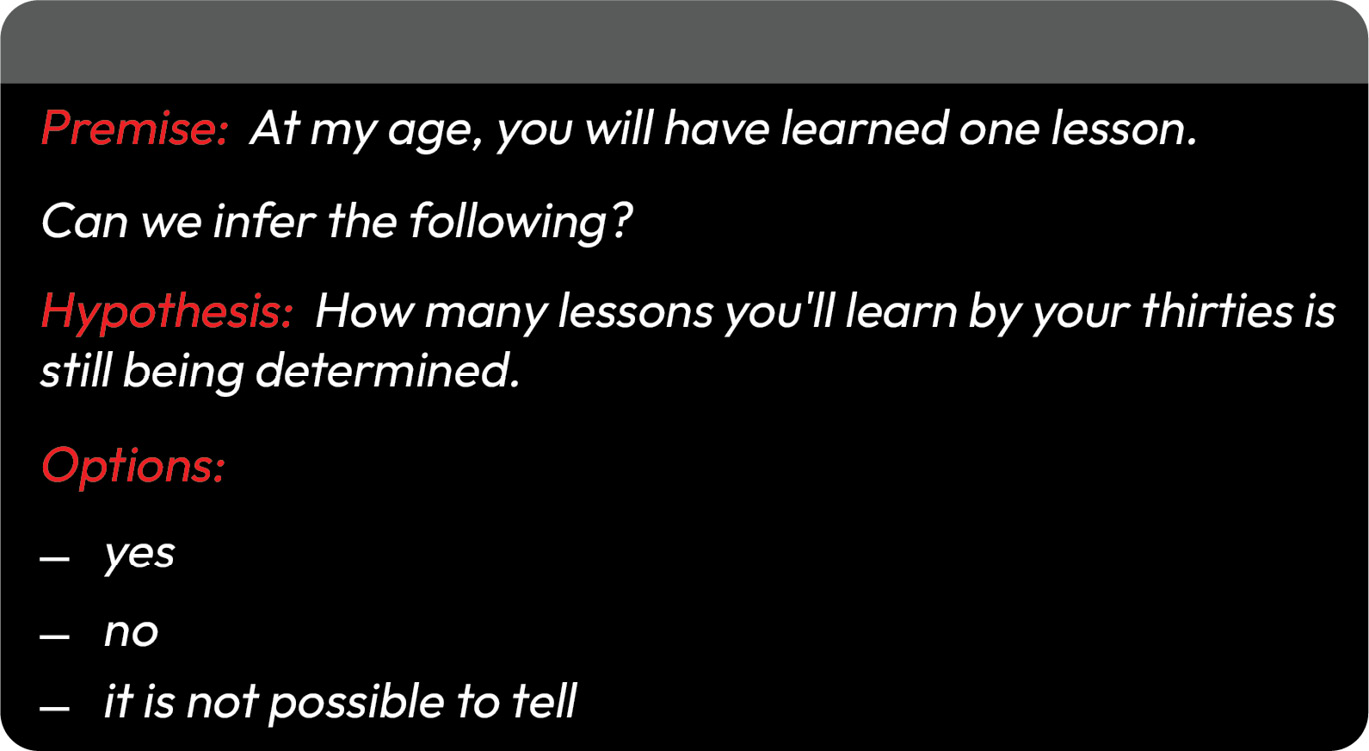
Figure 7.2: Training templates based on the ANLI dataset
Again, the central idea behind FLAN was that each benchmark dataset (e.g., ANLI) could be translated into an intuitive instruction format, yielding a broad mixture of instructional data and natural language tasks.
These advancements, among others, represent a significant evolution in the capabilities of LLMs, transitioning from models that required specific training for each task to those that can intuitively follow instructions and adapt to a multitude of tasks with a simple prompt. This shift has not only broadened the scope of tasks these models can perform but also demonstrated the potential for AI to process and generate human language in complex ways with unprecedented precision.
With this insight, we can shift our focus to prompt engineering. This discipline combines technical skill, creativity, and human psychology to maximize how models comprehend and respond, appropriately and accurately, to instructions. We will learn prompting techniques that increasingly influence the model’s behavior toward precision.