



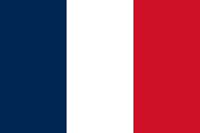


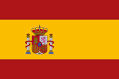




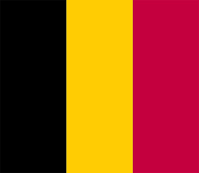
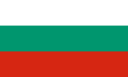
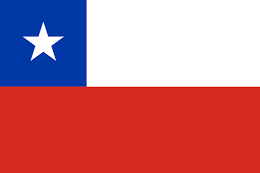
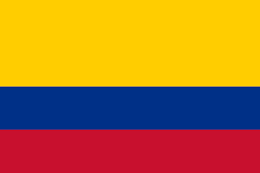
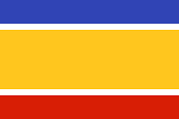

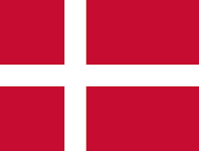



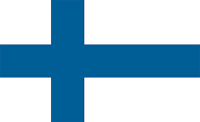

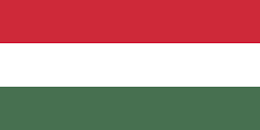






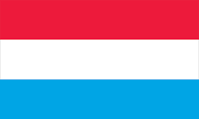
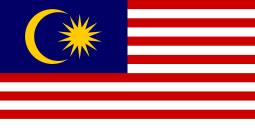

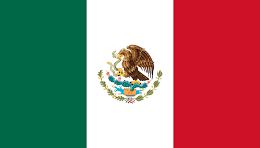

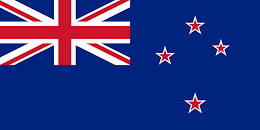







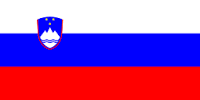




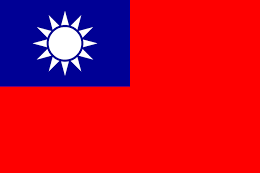



The Apollo community has built a machine-learning based auto-calibration system for autonomous driving vehicles. By August 2018, the system had been tested on more than two thousand hours with around ten thousands kilometers’ (6,213 miles) road tests and has proven to be effective. The system is automated and intelligent, due to which, it is suitable for mass-scale self-driving vehicle deployment.
Following are the main issues that the current system faces:
The auto-calibration system depends on the Apollo control module, which consists of an offline model and online learning algorithm
First, a calibration table is generated based on human driving data that best reflects vehicle longitudinal performance at the time of driving. It performs three functions:
The online algorithm updates the offline table based on real-time feedback in self-driving mode. It tries to best match the current vehicle dynamics based on offline model established from manual driving data. It performs the following functions:
To know more details on how this model works and helps to solve the manual calibration problem, check out their published paper: Baidu Apollo Auto-Calibration System - An Industry-Level Data-Driven and Learning based Vehicle Longitude Dynamic Calibrating Algorithm.
Apollo 11 source code: A small step for a woman, and a huge leap for ‘software engineering’
Baidu open sources ApolloScape and collaborates with Berkeley DeepDrive to further machine learning in automotives
Tesla is building its own AI hardware for self-driving cars