- An MAB is actually a slot machine, a gambling game played in a casino where you pull the arm (lever) and get a payout (reward) based on a randomly generated probability distribution. A single slot machine is called a one-armed bandit and, when there are multiple slot machines it is called multi-armed bandits or k-armed bandits.
- An explore-exploit dilemma arises when the agent is not sure whether to explore new actions or exploit the best action using the previous experience.
- The epsilon is used to for deciding whether the agent should explore or exploit actions with 1-epsilon we choose best action and with epsilon we explore new action.
- We can solve explore-exploit dilemma using a various algorithm such epsilon-greedy policy, softmax exploration, UCB, Thompson sampling.
- The UCB algorithm helps us in selecting the best arm based on a confidence interval.
- In Thomson sampling...




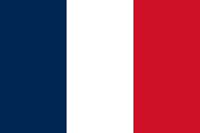


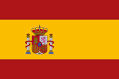




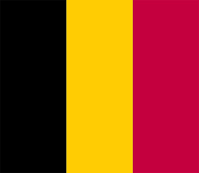
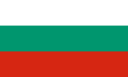
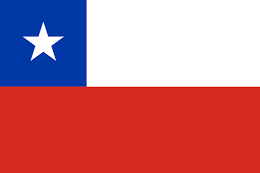
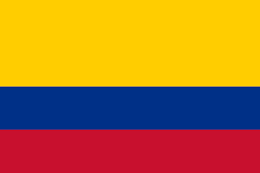
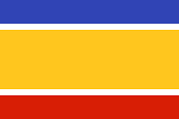

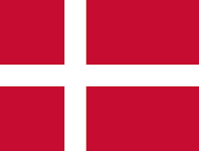



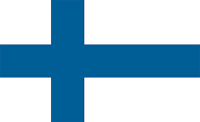

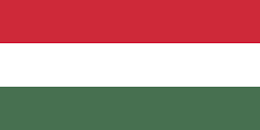






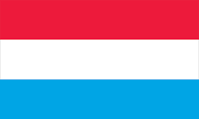
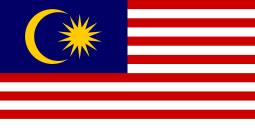

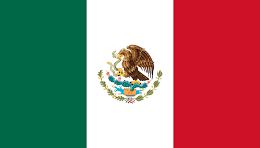

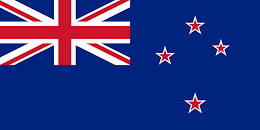







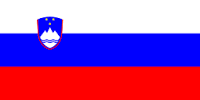




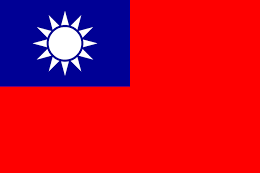


