The last variation of Q-learning architecture that we shall implement is the Dueling network architecture (https://arxiv.org/abs/1511.06581). As the name might suggest, here, we figuratively make a neural network duel with itself using two separate estimators for the value of a state and the value of a state-action pair. You will recall from earlier in this chapter that we estimated the quality of a state-action pairs using a single stream of convolutional and densely connected layers. However, we can actually split up the Q-value function into a sum of two separate terms. The reason behind this segregated architecture is to allow our model to separately learn states that may or may not be valuable, without having to specifically learn the effect of each action that's performed at each state:
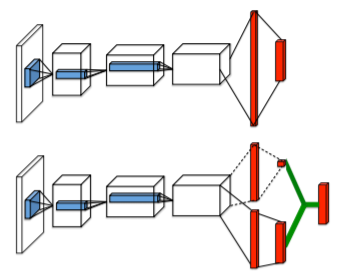
At the top of the preceding diagram, we can see the...