Spark standalone mode
If you have a set of machines without any existing cluster management software, you can deploy Spark over SSH with some handy scripts. This method is known as standalone mode in the Spark documentation at http://spark.apache.org/docs/latest/spark-standalone.html. An individual master and worker can be started by sbin/start-master.sh
and sbin/start-slaves.sh
, respectively. The default port for the master is 8080. As you likely don't want to go to each of your machines and run these commands by hand, there are a number of helper scripts in bin/
to help you run your servers.
A prerequisite for using any of the scripts is having password less SSH access set up from the master to all of the worker machines. You probably want to create a new user for running Spark on the machines and lock it down. This book uses the username sparkuser
. On your master, you can run ssh-keygen
to generate the SSH keys and make sure that you do not set a password. Once you have generated the key, add the public one (if you generated an RSA key, it would be stored in ~/.ssh/id_rsa.pub
by default) to ~/.ssh/authorized_keys2
on each of the hosts.
Tip
The Spark administration scripts require that your usernames match. If this isn't the case, you can configure an alternative username in your ~/.ssh/config
.
Now that you have the SSH access to the machines set up, it is time to configure Spark. There is a simple template in [filepath]conf/spark-env.sh.template[/filepath]
, which you should copy to [filepath]conf/spark-env.sh[/filepath]
.
You may also find it useful to set some (or all) of the environment variables shown in the following table:
Name |
Purpose |
Default |
|
This variable is used to point to math where Mesos lives. |
None |
|
This variable is used to point to where you extracted Scala. |
None, must be set |
|
This variable states the IP address for the master to listen on and the IP address for the workers to connect to. |
The result of running hostname |
|
This variable states the port |
|
|
This variable states the port |
|
|
This variable states the number of cores to use. |
All of them |
|
This variable states how much memory to use. |
Max of (system memory-1 GB, 512 MB) |
|
This variable states what port |
|
|
This variable states what port |
|
|
This variable states where to store files from the worker. |
|
Once you have completed your configuration, it's time to get your cluster up and running. You will want to copy the version of Spark and the configuration you have built to all of your machines. You may find it useful to install pssh
, a set of parallel SSH tools, including pscp
. The pscp
tool makes it easy to scp
to a number of target hosts, although it will take a while, as shown here:
pscp -v -r -h conf/slaves -l sparkuser ../opt/spark ~/
If you end up changing the configuration, you need to distribute the configuration to all of the workers, as shown here:
pscp -v -r -h conf/slaves -l sparkuser conf/spark-env.sh /opt/spark/conf/spark-env.sh
Tip
If you use a shared NFS on your cluster, while by default Spark names log files and similar with shared names, you should configure a separate worker directory; otherwise, they will be configured to write to the same place. If you want to have your worker directories on the shared NFS, consider adding 'hostname'
, for example SPARK_WORKER_DIR=~/work-'hostname'
.
You should also consider having your log files go to a scratch directory for performance.
Now you are ready to start the cluster and you can use the sbin/start-all.sh
, sbin/start-master.sh
, and sbin/start-slaves.sh
scripts. It is important to note that start-all.sh
and start-master.sh
both assume that they are being run on the node, which is the master for the cluster. The start scripts all daemonize, and so you don't have to worry about running them on a screen.
ssh master bin/start-all.sh
If you get a class not found error stating java.lang.NoClassDefFoundError: scala/ScalaObject
, check to make sure that you have Scala installed on that worker host and that the SCALA_HOME
is set correctly.
Tip
The Spark scripts assume that your master has Spark installed in the same directory as your workers. If this is not the case, you should edit bin/spark-config.sh
and set it to the appropriate directories.
The commands provided by Spark to help you administer your cluster are given in the following table. More details are available on the Spark website at http://spark.apache.org/docs/latest/spark-standalone.html#cluster-launch-scripts.
Command |
Use |
|
This command runs the provided command on all of the worker hosts. For example, |
|
This command starts the master and all of the worker hosts. This command must be run on the master. |
|
This command starts the master host. This command must be run on the master. |
|
This command starts the worker hosts. |
|
This command starts a specific worker. |
|
This command stops master and workers. |
|
This command stops the master. |
|
This command stops all the workers. |
You now have a running Spark cluster. There is a handy Web UI on the master running on port 8080 you should go and visit, and on all of the workers on port 8081. The Web UI contains helpful information such as the current workers, and current and past jobs.
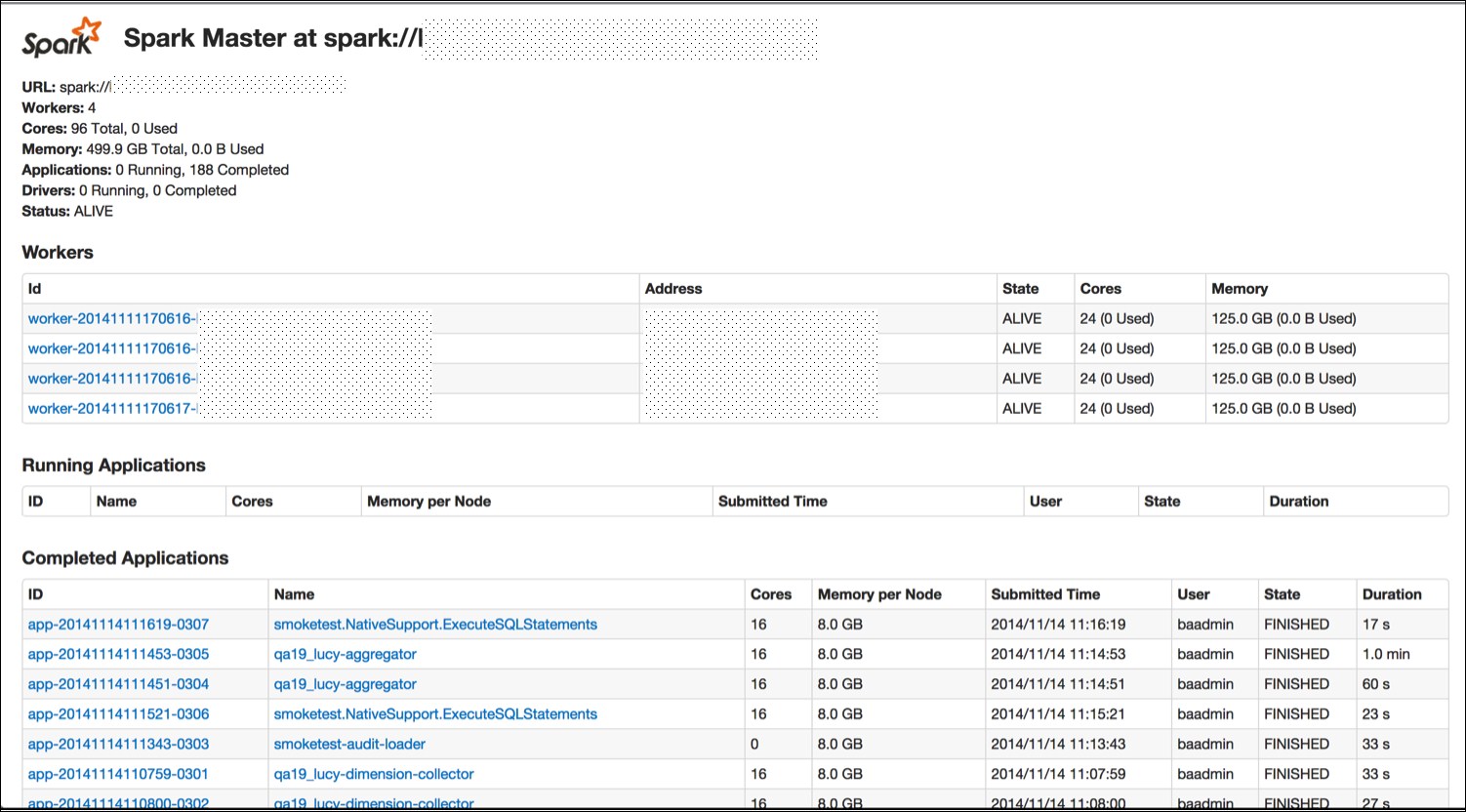
Now that you have a cluster up and running, let's actually do something with it. As with the single host example, you can use the provided run script to run the Spark commands. All of the examples listed in examples/src/main/scala/spark/org/apache/spark/examples/
take a parameter, master
, which points them to the master. Assuming that you are on the master host, you could run them with this command:
./run-example GroupByTest spark://'hostname':7077
Tip
If you run into an issue with java.lang.UnsupportedClassVersionError
, you may need to update your JDK or recompile Spark if you grabbed the binary version. Version 1.1.0 was compiled with JDK 1.7 as the target. You can check the version of the JRE targeted by Spark with the following commands:
java -verbose -classpath ./core/target/scala- 2.9.2/classes/ spark.SparkFiles |head -n 20
Version 49 is JDK1.5, Version 50 is JDK1.6, and Version 60 is JDK1.7.
If you can't connect to localhost
, make sure that you've configured your master (spark.driver.port
) to listen to all of the IP addresses (or if you don't want to replace localhost
with the IP address configured to listen to). More port configurations are listed at http://spark.apache.org/docs/latest/configuration.html#networking.
If everything has worked correctly, you will see the following log messages output to stdout
:
13/03/28 06:35:31 INFO spark.SparkContext: Job finished: count at GroupByTest.scala:35, took 2.482816756 s2000