In many common practices, data is limited. Training a deep neural network such as ConvNet, which has millions of parameters on a small set of data, can lead to overfitting. To avoid such issues, a common practice is to leverage an existing deep neural network that was trained on a much larger dataset, such as ImageNet (1.2 million labeled images), and fine-tune it on the smaller dataset at hand, which is not drastically different; that is, to continue to train the existing network using this new and smaller dataset. As we have discussed, one of the advantages of deep learning networks is that the first few layers often represent more general patterns mined from the data. Fine-tuning essentially leverages the common knowledge learned from a large pool of data and applies it to a specific area/application. For example, the first few layers in ConvNet may capture universal...




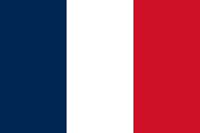


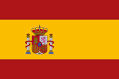



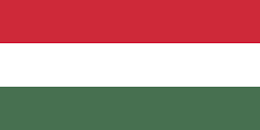

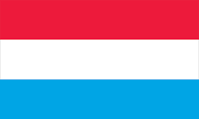





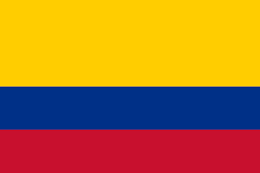
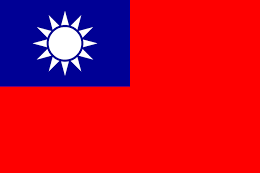
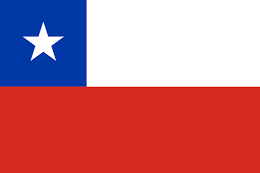



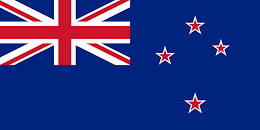
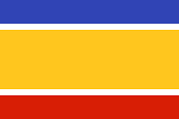
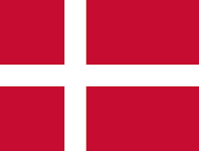
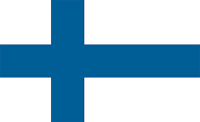







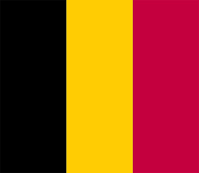

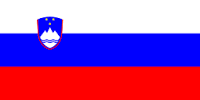





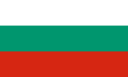


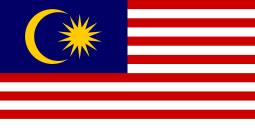



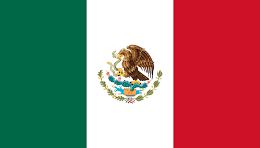
