Feature extraction
Given a dataset of face regions, we can use feature extraction to obtain the feature vector, which gives us the most important information from the expression. The following figure shows the process that we use in our implementation to extract features vectors:
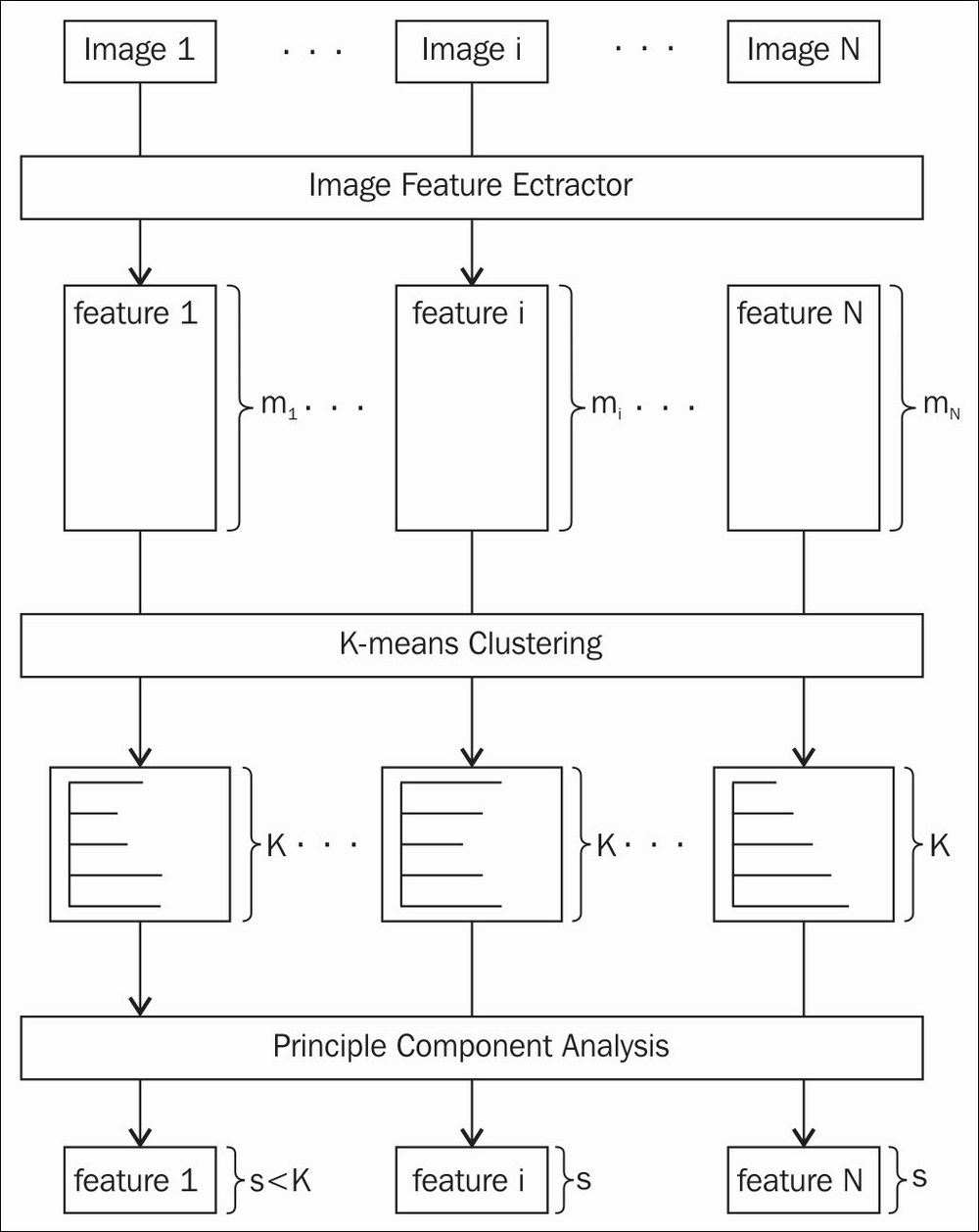
The feature extraction process
In order to understand this chapter, you need to understand that the feature representation of the expression image is the distribution of image features over k clusters (k = 1000 in our implementation). We have implemented a few common types of features that are supported in OpenCV, such as SIFT, SURF, and some advanced features, such as DENSE-SIFT, KAZE, DAISY. Since these image features are computed at image key points such as corners, except for DENSE cases, the number of image features can vary between images. However, we want to have a fixed feature size for every image to perform classification, since we will apply machine learning classification techniques later. It is important...