BigQuery is an extremely powerful data warehousing solution provided by Google. Pandas can directly connect to BigQuery and bring your data to a Python environment for further analysis.
The following is an example of reading a dataset from BigQuery:
pd.read_gbq("SELECT urban_area_code, geo_code, name, area_type, area_land_meters
FROM `bigquery-public-data.utility_us.us_cities_area` LIMIT 5", project_id, dialect = "standard")
Take a look at the following output:
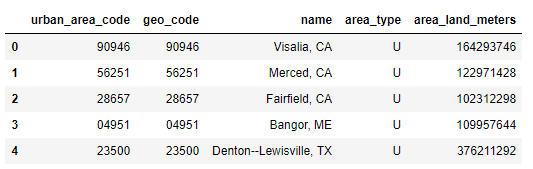
Output of read_gbq
The read_gbq() function accepts the query and the Google Cloud project-id (which serves as a key) so that it can access the database and bring out the data. The dialect argument takes care of the SQL syntax to be used: BigQuery's legacy SQL dialect or the standard SQL dialect. In addition, there are arguments that allow the index column to be set...