Congratulations! We've made it to the final chapter. We've come a long way. We started off with meta learning fundamentals and then we saw several one-shot learning algorithms such as siamese, prototypical, matching, and relation networks. Later, we also saw how NTM stores and retrieves information. Going ahead, we saw interesting meta learning algorithms such as MAML, Reptile, and Meta-SGD. We saw how these algorithms find an optimal initial parameter. Now, we'll see some of the recent advancements in meta learning. We'll learn about how task agnostic meta learning is used for reducing task bias in meta learning and how meta learning is used in the imitation learning system. Then, we'll see how can we apply MAML in an unsupervised learning setting using the CACTUs algorithm. Later, we'll learn about a deep meta...




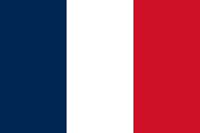


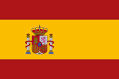



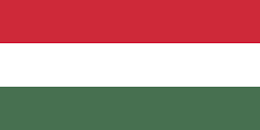

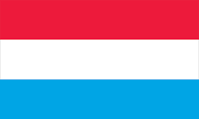





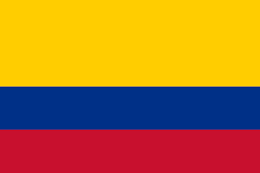
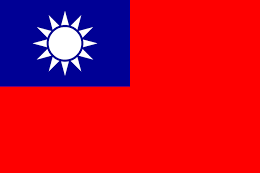
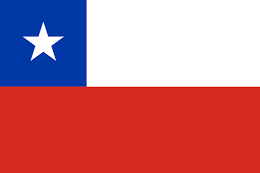



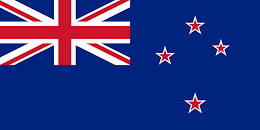
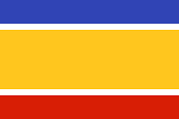
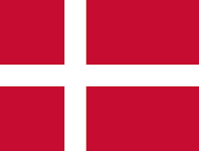
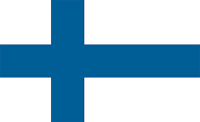







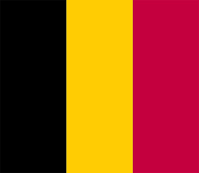

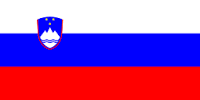





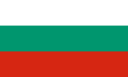


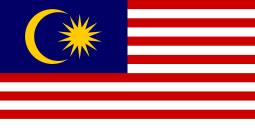



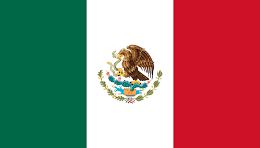
