There are several tasks that are crucial for the success of a machine learning model when applied to solve a given business problem, for example:
- Data pre-processing
- Feature engineering
- Model selection
- Optimization of the model hyperparameters
- Analysis of the model results
These tasks were usually performed more or less manually by experts in the field. In recent years, there has been a growing interest in democratizing machine learning, allowing for non-experts (sometimes called citizen data scientists) to use, improve, and apply machine learning to concrete problems. Automated Machine Learning (AutoML) targets that specific need.
In general, the building process of a new model can be described as in the following diagram:
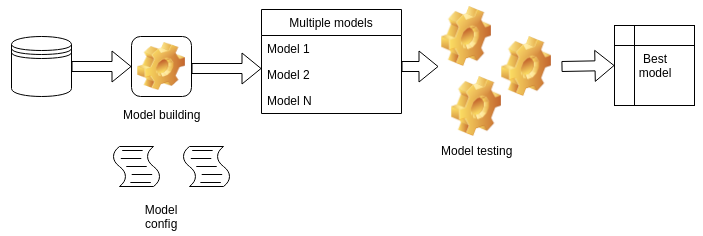
Following is the process for building of new model:
- Input data is pre-processed and used to build the best model features
- Based...