Exploring RNNs
In this section, we'll explore RNNs. Some background information will start us off, and then we will look at a motivating weather modeling problem. We'll also implement and train an RNN in TensorFlow.
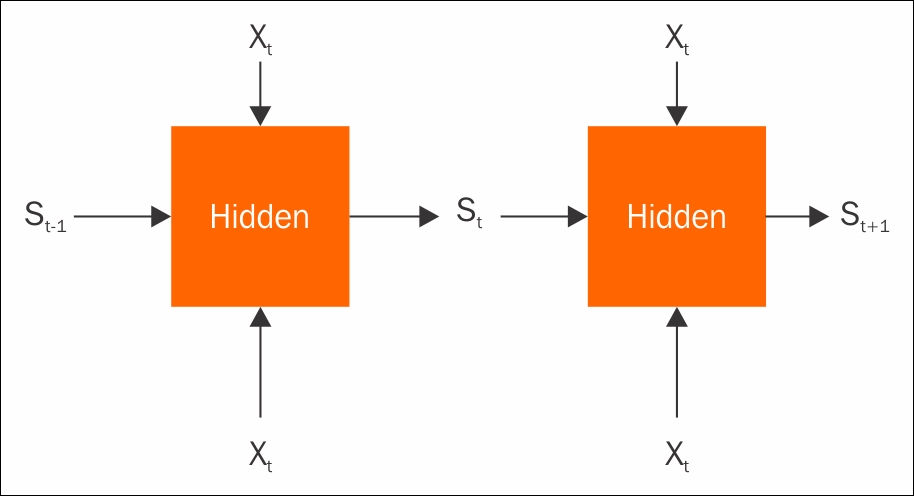
In a typical model, you have some X input features and some Y output you want to predict. We usually consider our different training samples as independent observations. So, the features from data point one shouldn't impact the prediction for data point two. But what if our data points are correlated? The most common example is that each data point, Xt, represents features collected at time t. It's natural to suppose that the features at time t and time t+1 will both be important to the prediction at time t+1. In other words, history matters.
Now, when modeling, you could just include twice as many input features, adding the previous time step to the current ones, and computing twice as many input weights. But, if you're going through all the effort of...