As machine learning has progressed over the last few years, I have come across many ways to categorize the different types of learning. Recently, at the NeurIPS 2018 conference in Montreal, Canada, Dr. Alex Graves shared information about the different types of learning, shown in Figure 7.1:
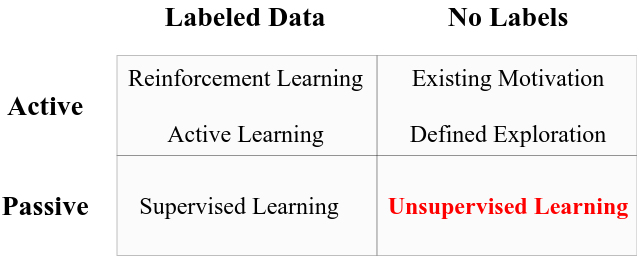
Such efforts at categorization are very useful today when there are many learning algorithms being studied and improved. The first row depicts active learning, which means that there is a sense of interaction between the learning algorithm and the data. For example, in reinforcement learning and active learning operating over labeled data, the reward policies can inform what type of data the model will read in the following iterations. However, traditional supervised learning, which is what we have studied so far, involves no interaction with the data source and instead assumes that the dataset is fixed and that...