Evaluating Classification Models
Classification models require a bunch of different metrics to be thoroughly evaluated, unlike regression models. Here, we don't have something as intuitive as R Squared. Moreover, the performance requirements completely change based on a specific use case. Let's take a brief look at the various metrics that we already studied in Chapter 3, Introduction to Supervised Learning, for classification.
Confusion Matrix and Its Derived Metrics
The first basis for studying model performance for classification algorithms starts with a confusion matrix. A confusion matrix is a simple representation of the distribution of predictions of each class across the actuals of each class:
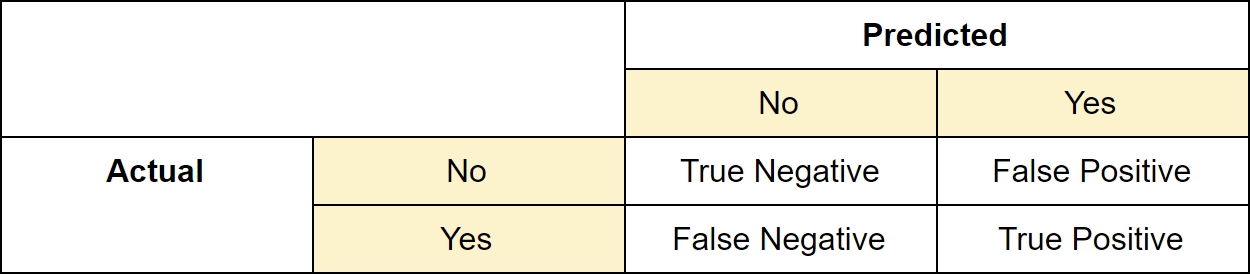
Figure 5.3: Confusion matrix
The previous table is a simple representation of a confusion matrix. Here, we assume that the Yes class is labelled Positive. When the actual value of a given sample is Yes and it is correctly predicted as Positive, we define it as True Positive, whereas, if the actual...