



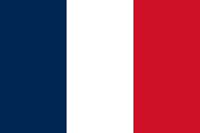


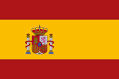



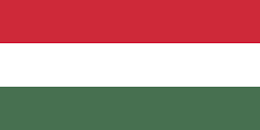

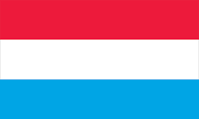





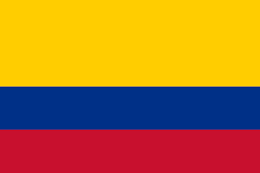
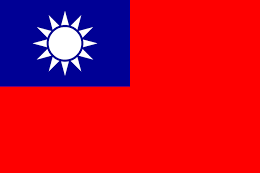
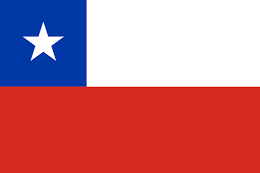



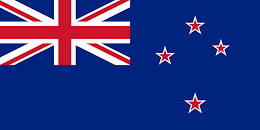
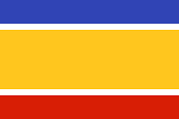
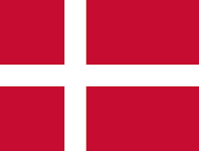
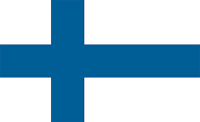







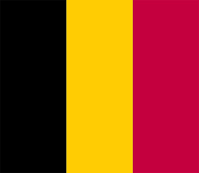

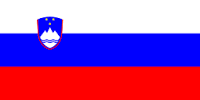





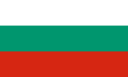


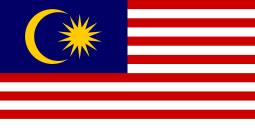



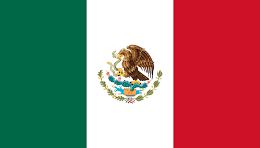

A few days ago, Alibaba Cloud announced the release of Mars, its tensor-based framework for large-scale data computation. Mars tensor provides a familiar interface like Numpy, which is a popular tool for most of the Python users such as mathematicians, engineers, etc. and the ones working in core scientific computing. Mars can also scale into a single machine, and scale out to a cluster with hundreds of machines.
Users can simply install Mars tensor with the following code:
import mars.tensor as mta = mt.random.rand(1000, 2000)(a + 1).sum(axis=1).execute()
According to a Medium post by Synced, “Mars can simply tile a large tensor into small chunks and describe the inner computation with a directed graph, enabling the running of parallel computation on a wide range of distributed environments, from a single machine to a cluster comprising thousands of machines.”
Xuye Qin, Alibaba Cloud Senior Engineer, bragged about Mars’ performance by stating, “Mars can complete the computation on a 2.25T-size matrix and a 2.25T-size matrix multiplication in two hours.”
Unlike NumPy, Mars provides users with the ability to run matrix computation at a very large-scale. Alibaba developers carried out a simple experiment to test Mars’ performance. According to the graph below where NumPy (represented by a red cross at the upper left) lags far behind Mars tensors, which is successful in achieving ideal performance values.
Source: Medium
Mars supports a subset of NumPy interfaces, which include:
To know more about Mars in detail, visit its official GitHub page.
NumPy drops Python 2 support. Now you need Python 3.5 or later
Google researchers introduce JAX: A TensorFlow-like framework for generating high-performance code from Python and NumPy machine learning programs
Introducing numpywren, a system for linear algebra built on a serverless architecture