



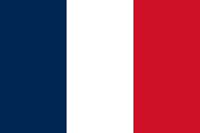


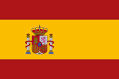



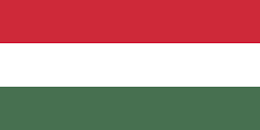

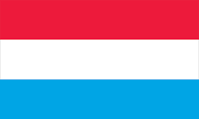





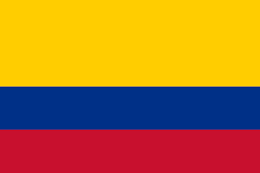
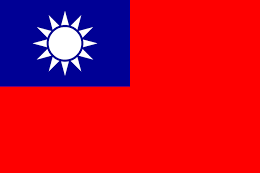
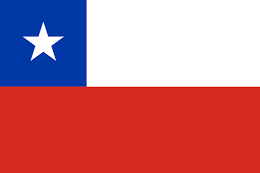



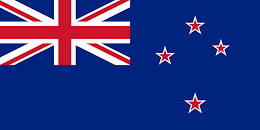
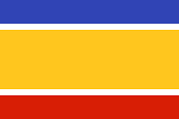
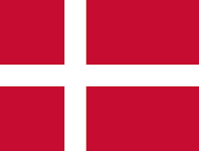
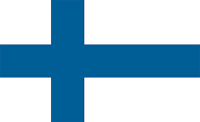







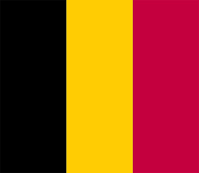

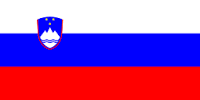





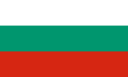


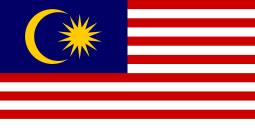



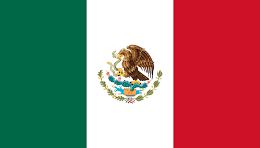

In this article by Shantanu Kumar, author of the book, Clojure High Performance Programming - Second Edition, we learn how Clojure is a safe, functional programming language that brings great power and simplicity to the user. Clojure is also dynamically and strongly typed, and has very good performance characteristics. Naturally, every activity performed on a computer has an associated cost. What constitutes acceptable performance varies from one use-case and workload to another. In today's world, performance is even the determining factor for several kinds of applications. We will discuss Clojure (which runs on the JVM (Java Virtual Machine)), and its runtime environment in the light of performance, which is the goal of the book.
In this article, we will study the basics of performance analysis, including the following:
(For more resources related to this topic, see here.)
The performance requirements and priority vary across the different kinds of use cases. We need to determine what constitutes acceptable performance for the various kinds of use cases. Hence, we classify them to identify their performance model. When it comes to details, there is no sure shot performance recipe of any kind of use case, but it certainly helps to study their general nature. Note that in real life, the use cases listed in this section may overlap with each other.
The performance of user facing applications is strongly linked to the user's anticipation. Having a difference of a good number of milliseconds may not be perceptible for the user but at the same time, a wait for more than a few seconds may not be taken kindly. One important element to normalize the anticipation is to engage the user by providing a duration-based feedback. A good idea to deal with such a scenario would be to start the task asynchronously in the background, and poll it from the UI layer to generate duration-based feedback for the user. Another way could be to incrementally render the results to the user to even out the anticipation.
Anticipation is not the only factor in user facing performance. Common techniques like staging or precomputation of data, and other general optimization techniques can go a long way to improve the user experience with respect to performance. Bear in mind that all kinds of user facing interfaces fall into this use case category—the Web, mobile web, GUI, command line, touch, voice-operated, gesture...you name it.
Non-trivial compute intensive tasks demand a proportional amount of computational resources. All of the CPU, cache, memory, efficiency and the parallelizability of the computation algorithms would be involved in determining the performance. When the computation is combined with distribution over a network or reading from/staging to disk, I/O bound factors come into play. This class of workloads can be further subclassified into more specific use cases.
A CPU bound computation is limited by the CPU cycles spent on executing it. Arithmetic processing in a loop, small matrix multiplication, determining whether a number is a Mersenne prime, and so on, would be considered CPU bound jobs. If the algorithm complexity is linked to the number of iterations/operations N, such as O(N), O(N2) and more, then the performance depends on how big N is, and how many CPU cycles each step takes. For parallelizable algorithms, performance of such tasks may be enhanced by assigning multiple CPU cores to the task. On virtual hardware, the performance may be impacted if the CPU cycles are available in bursts.
A memory bound task is limited by the availability and bandwidth of the memory. Examples include large text processing, list processing, and more. For example, specifically in Clojure, the (reduce f (pmap g coll)) operation would be memory bound if coll is a large sequence of big maps, even though we parallelize the operation using pmap here. Note that higher CPU resources cannot help when memory is the bottleneck, and vice versa. Lack of availability of memory may force you to process smaller chunks of data at a time, even if you have enough CPU resources at your disposal. If the maximum speed of your memory is X and your algorithm on single the core accesses the memory at speed X/3, the multicore performance of your algorithm cannot exceed three times the current performance, no matter how many CPU cores you assign to it. The memory architecture (for example, SMP and NUMA) contributes to the memory bandwidth in multicore computers. Performance with respect to memory is also subject to page faults.
A task is cache bound when its speed is constrained by the amount of cache available. When a task retrieves values from a small number of repeated memory locations, for example, a small matrix multiplication, the values may be cached and fetched from there. Note that CPUs (typically) have multiple layers of cache, and the performance will be at its best when the processed data fits in the cache, but the processing will still happen, more slowly, when the data does not fit into the cache. It is possible to make the most of the cache using cache-oblivious algorithms. A higher number of concurrent cache/memory bound threads than CPU cores is likely to flush the instruction pipeline, as well as the cache at the time of context switch, likely leading to a severely degraded performance.
An input/output (I/O) bound task would go faster if the I/O subsystem, that it depends on, goes faster. Disk/storage and network are the most commonly used I/O subsystems in data processing, but it can be serial port, a USB-connected card reader, or any I/O device. An I/O bound task may consume very few CPU cycles. Depending on the speed of the device, connection pooling, data compression, asynchronous handling, application caching, and more, may help in performance. One notable aspect of I/O bound tasks is that performance is usually dependent on the time spent waiting for connection/seek, and the amount of serialization that we do, and hardly on the other resources.
In practice, many data processing workloads are usually a combination of CPU bound, memory bound, cache bound, and I/O bound tasks. The performance of such mixed workloads effectively depends on the even distribution of CPU, cache, memory, and I/O resources over the duration of the operation. A bottleneck situation arises only when one resource gets too busy to make way for another.
The online transaction processing (OLTP) systems process the business transactions on demand. It can sit behind systems such as a user-facing ATM machine, point-of-sale terminal, a network-connected ticket counter, ERP systems, and more. The OLTP systems are characterized by low latency, availability, and data integrity. They run day-to-day business transactions. Any interruption or outage is likely to have a direct and immediate impact on the sales or service. Such systems are expected to be designed for resiliency rather than the delayed recovery from failures. When the performance objective is unspecified, you may like to consider graceful degradation as a strategy.
It is a common mistake to ask the OLTP systems to answer analytical queries; something that they are not optimized for. It is desirable of an informed programmer to know the capability of the system, and suggest design changes as per the requirements.
The online analytical processing (OLAP) systems are designed to answer analytical queries in short time. They typically get data from the OLTP operations, and their data model is optimized for querying. They basically provide for consolidation (roll-up), drill-down and slicing, and dicing of data for analytical purposes. They often use specialized data stores that can optimize the ad-hoc analytical queries on the fly. It is important for such databases to provide pivot-table like capability. Often, the OLAP cube is used to get fast access to the analytical data.
Feeding the OLTP data into the OLAP systems may entail workflows and multistage batch processing. The performance concern of such systems is to efficiently deal with large quantities of data, while also dealing with inevitable failures and recovery.
Batch processing is automated execution of predefined jobs. These are typically bulk jobs that are executed during off-peak hours. Batch processing may involve one or more stages of job processing. Often batch processing is clubbed with work-flow automation, where some workflow steps are executed offline. Many of the batch processing jobs work on staging of data, and on preparing data for the next stage of processing to pick up.
Batch jobs are generally optimized for the best utilization of the computing resources. Since there is little to moderate the demand to lower the latencies of some particular subtasks, these systems tend to optimize for throughput. A lot of batch jobs involve largely I/O processing and are often distributed over a cluster. Due to distribution, the data locality is preferred when processing the jobs; that is, the data and processing should be local in order to avoid network latency in reading/writing data.
We learned about the basics of what it is like to think more deeply about performance. The performance of Clojure applications depend on various factors. For a given application, understanding its use cases, design and implementation, algorithms, resource requirements and alignment with the hardware, and the underlying software capabilities, is essential.
Further resources on this subject: