In the previous recipe, we learned about some of the basic concepts of machine learning. We demonstrated how a classifier can be built by collecting samples of the different classes of interest. However, for the approach that was considered in this previous recipe, training a classifier simply consists of storing all the samples' representations. From there, the label of any new instance can be predicted by looking at the closest (nearest neighbor) labeled point. For most machine learning methods, training is a relatively iterative process, during which machinery is built by looping over the samples. The performance of the classifier produced gradually improves as more samples are presented. Learning eventually stops when a certain performance criterion is reached, or when no more improvements can be obtained from...




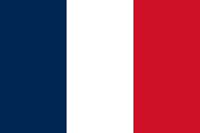


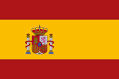



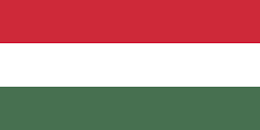

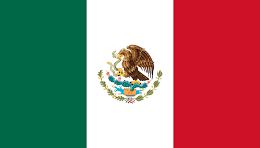


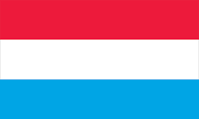



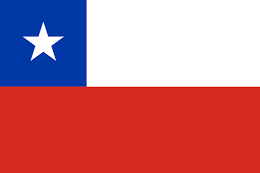


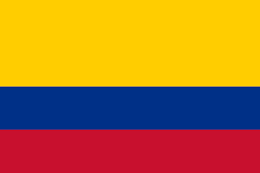
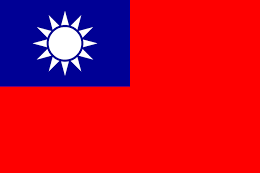


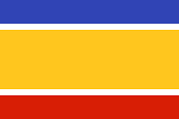
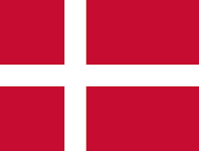
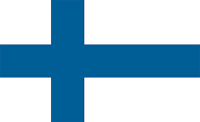



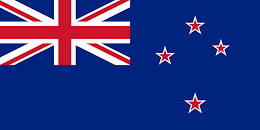





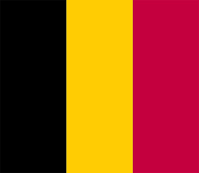

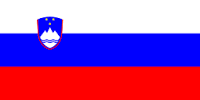




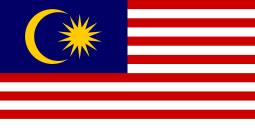


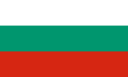


