Dealing with missing values can be a little tricky as there's a number of ways to approach the task. We've already seen in the section on descriptive statistics that there're missing values. First of all, let's get a full accounting of the missing quantity by feature, then we shall discuss how to deal with them. What I'm going to demonstrate in the following is how to put the count by feature into a dataframe that we can explore within RStudio:
na_count <-
sapply(gettysburg, function(y)
sum(length(which(is.na(
y
)))))
na_df <- data.frame(na_count)
View(na_df)
The following is a screenshot produced by the preceding code, after sorting the dataframe by descending count:
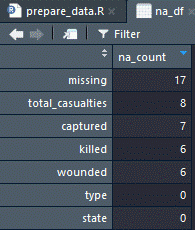
You can clearly see the count of missing by feature with the most missing is ironically named missing with a total of 17 observations.
So what should we do here or, more appropriately, what can we do here? There're several choices:
- Do nothing: However, some R functions will omit NAs and some functions will fail and produce an error.
- Omit all observations with NAs: In massive datasets, they may make sense, but we run the risk of losing information.
- Impute values: They could be something as simple as substituting the median value for the missing one or creating an algorithm to impute the values.
- Dummy coding: Turn the missing into a value such as 0 or -999, and code a dummy feature where if the feature for a specific observation is missing, the dummy is coded 1, otherwise, it's coded 0.
I could devote an entire chapter, indeed a whole book on the subject, delving into missing at random and others, but I was trained—and, in fact, shall insist—on the latter method. It's never failed me and the others can be a bit problematic. The benefit of dummy coding—or indicator coding, if you prefer—is that you don't lose information. In fact, missing-ness might be an essential feature in and of itself.
So, here's an example of how I manually code a dummy feature and turn the NAs into zeroes:
gettysburg$missing_isNA <-
ifelse(is.na(gettysburg$missing), 1, 0)
gettysburg$missing[is.na(gettysburg$missing)] <- 0
The first iteration of code creates a dummy feature for the missing feature and the second changes any NAs in missing to zero. In the upcoming section, where the dataset is fully processed (treated), the other missing values will be imputed.