Understanding the ML project life cycle
The following are the major steps in an ML project:
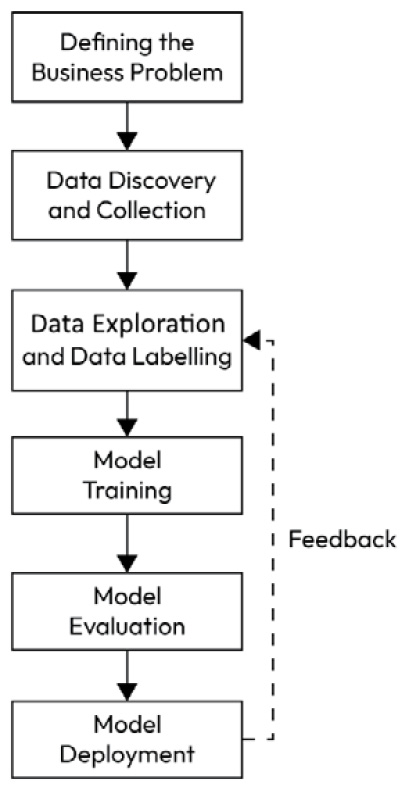
Figure 1.1 – ML project life cycle diagram
Let’s look at them in detail.
Defining the business problem
The first step in every ML project is to understand the business problem and define clear goals that can be measured at the end of the project.
Data discovery and data collection
In this step, you identify and gather potential data sources that may be relevant to your project’s objectives. This involves finding datasets, databases, APIs, or any other sources that may contain the data needed for your analysis and modeling.
The goal of data discovery is to understand the landscape of available data and assess its quality, relevance, and potential limitations.
Data discovery can also involve discussions with domain experts and stakeholders to identify what data is essential for solving business problems or achieving the project’s goals.
After identifying various sources for data, data engineers will develop data pipelines to extract and load the data to the target data lake and perform some data preprocessing tasks such as data cleaning, de-duplication, and making data readily available to ML engineers and data scientists for further processing.
Data exploration
Data exploration follows data discovery and is primarily focused on understanding the data, gaining insights, and identifying patterns or anomalies.
During data exploration, you may perform basic statistical analysis, create data visualizations, and conduct initial observations to understand the data’s characteristics.
Data exploration can also involve identifying missing values, outliers, and potential data quality issues, but it typically does not involve making systematic changes to the data.
During data exploration, you assess the available labeled data and determine whether it’s sufficient for your ML task. If you find that the labeled data is small and insufficient for model training, you may identify the need for additional labeled data.
Data labeling
Data labeling involves acquiring or generating more labeled examples to supplement your training dataset. You may need to manually label additional data points or use programming techniques such as data augmentation to expand your labeled dataset. The process of assigning labels to data samples is called data annotation or data labeling.
Most of the time, it is too expensive or time-consuming to outsource the manual data labeling task. Also, data is often not allowed to be shared with external third-party organizations due to data privacy. So, automating the data labeling process with an in-house development team using Python helps to label the data quickly and at an affordable cost.
Most of the data science books available on the market are lacking information about this important step. So, this book aims to address the various methods to programmatically label data using Python as well as the annotation tools available on the market.
After obtaining a sufficient amount of labeled data, you proceed with traditional data preprocessing tasks, such as handling missing values, encoding features, scaling, and feature engineering.
Model training
Once the data is adequately prepared, then that dataset is fed into the model by ML engineers to train the model.
Model evaluation
After the model is trained, the next step is to evaluate the model on a validation dataset to see how good the model is and avoid bias and overfitting.
You can evaluate the model’s performance using various metrics and techniques and iterate on the model-building process as needed.
Model deployment
Finally, you deploy your model into production and monitor for continuous improvement using ML Operations (MLOps). MLOps aims to streamline the process of taking ML models to production and maintaining and monitoring them.
In this book, we will focus on data labeling. In a real-world project, the datasets that sources provide us with for analytics and ML are not clean and not labeled. So, we need to explore unlabeled data to understand correlations and patterns and help us define the rules for data labeling using Python labeling functions. Data exploration helps us to understand the level of cleaning and transformation required before starting data labeling and model training.
This is where Python helps us to explore and perform a quick analysis of raw data using various libraries (such as Pandas, Seaborn, and ydata-profiling libraries), otherwise known as EDA.