Auxiliary classifier GAN (ACGAN)
ACGAN is similar in principle to the Conditional GAN (CGAN) that we discussed in the previous chapter. We're going to compare both CGANand ACGAN. For both CGAN and ACGAN, the generator inputs are noise and its label. The output is a fake image belonging to the input class label. For CGAN, the inputs to the discriminator are an image (fake or real) and its label. The output is the probability that the image is real. For ACGAN, the input to the discriminator is an image, whilst the output is the probability that the image is real and its class label. Following figure highlights the difference between CGAN and ACGAN during generator training:
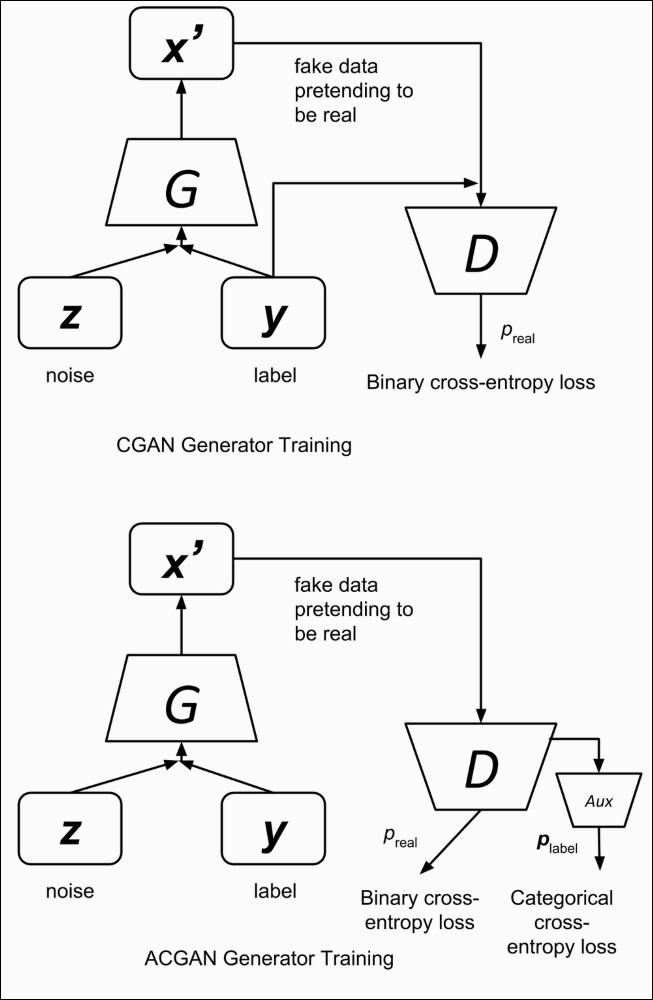
Figure 5.3.1: CGAN vs. ACGAN generator training. The main difference is the input and output of the discriminator.
Essentially, in CGAN we feed the network with side information (label). In ACGAN, we try to reconstruct the side information using an auxiliary class decoder network. ACGAN argued that forcing the network to...