



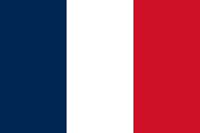


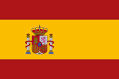



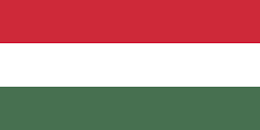

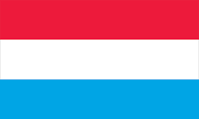





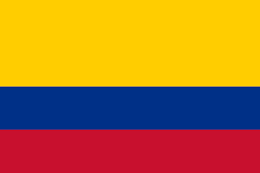
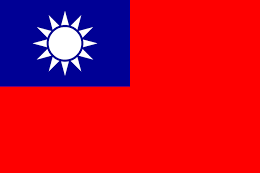
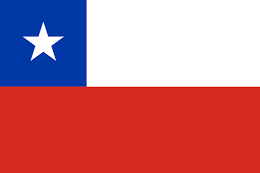



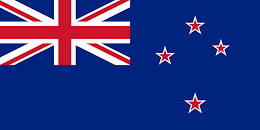
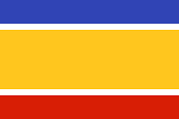
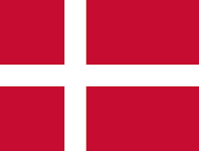
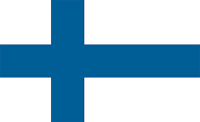







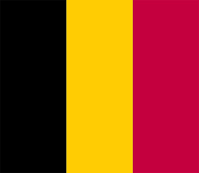

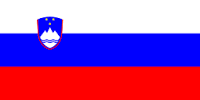





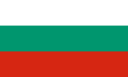


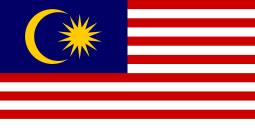



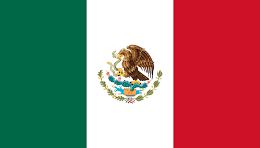

Dive deeper into the world of AI innovation and stay ahead of the AI curve! Subscribe to our AI_Distilled newsletter for the latest insights. Don't miss out – sign up today!
For many, the world of stock trading can seem like a puzzle, but it operates on some core principles. People in the stock market use different strategies to decide when to buy or sell. One popular approach is observing market trends and moving with them, much like a sailor adjusting sails to the wind. Another strategy believes that if prices swing too high or too low, they'll eventually return to their usual state - akin to a pendulum finding its center. Some traders have a straightforward method: buy when things look good and sell when they don't, as simple as following a recipe. And then there are those who patiently wait for prices to break past their usual limits, similar to a birdwatcher waiting for the perfect moment to spot a rare bird. This guide aims to unpack each of these strategies in an easy-to-understand manner, offering insights into the foundational methods of stock trading. A few strategies which we are going to implement is discussed below.
Trend Following capitalizes on the market's momentum in a specific direction, often using tools such as moving averages, MACD, and the ADX to decipher potential gains. In contrast, Mean Reversion operates on the belief that prices or returns gravitate back to their historical average; tools like Bollinger Bands and RSI become crucial in identifying overextended assets. Momentum (or Trend Momentum) takes a similar trajectory but focuses on amplifying returns by purchasing high-performing assets and shedding those underperforming ones, with instruments like the Rate of Change (ROC) or Relative Strength acting as key metrics. Lastly, Breakout Trading is about capitalizing on decisive market moves, wherein a trader either buys an asset breaking past a resistance or sells one dropping below a support level. Among these strategies, the moving average crossover technique stands out as a pivotal tool, especially for those keen on trend following, capturing moments when short-term and long-term averages intersect to suggest potential market shifts.
Understanding the stock market isn't always straightforward. Many people use different methods to figure out when to buy or sell stocks. Some observe general market movements and go with the flow, while others believe prices usually return to a familiar middle ground after big shifts. There's also the group that buys when things look promising and sells when they seem less favorable. And some? They wait for those standout moments when stock prices move outside their usual range. To get a clearer picture of these methods, modern tools like CHATGPT's data analysis have become handy. They sift through loads of data to help people sharpen their trading tactics. This guide will shed light on these strategies and how tools like CHATGPT make things simpler in the fast-moving world of stocks.
You can access the data here (Link)
As a Stock Analyst specializing in trend-following strategies, develop a concise report on APPLE stock using the moving average crossover technique, focusing on the 50-day and 200-day averages. Plot the moving averages to identify "Buy" or "Sell" signals when they cross and backtest these signals against historical data to gauge performance. Present your findings in a visually accessible format with charts for clarity.
Response: Let's apply the trend-following strategy using the moving average crossover technique on this new dataset. We'll compute the short-term (50-day) and long-term (200-day) moving averages, generate buy/sell signals based on the crossovers, and then backtest the strategy.
After backtesting the trend-following strategy using moving average crossovers on the new dataset:
It seems there was a significant loss during the period using this strategy on the dataset. This could be due to the specific characteristics of the stock during this timeframe, the chosen moving average windows (50-day and 200-day), or other market factors.
To gain a better understanding, let's visualize the stock price, short-term moving average, and long-term moving average with buy and sell points.
The graph visualizes the stock's closing price (in blue), the short-term 50-day moving average (in green), and the long-term 200-day moving average (in red). Buy signals are represented by green upward-pointing triangles, while sell signals are shown as red downward-pointing triangles.
Observations:
As a Stock Analyst specializing in mean reversion strategies, develop a succinct report on APPLE stock. Analyze periods when the stock deviates significantly from its historical average and identify potential opportunities where the stock may revert to its mean. Use both statistical metrics and visual charts to highlight these deviations. Ensure to backtest the identified mean reversion points against historical data to assess the strategy's effectiveness. Summarize your findings in a visually engaging format with relevant charts.
Response: A mean reversion strategy assumes that prices will tend to revert to their historical mean over time. One common tool used for a mean reversion strategy is the Bollinger Bands, which envelop the price structure of a stock to describe a trading band.
Bollinger Bands Mean Reversion Strategy:
Bollinger Bands consist of:
Trading Rules:
Let's apply the Bollinger Bands mean reversion strategy to the dataset, compute the buy/sell signals, and then backtest the strategy. After backtesting the Bollinger Bands-based mean reversion strategy on the dataset:
The outcome suggests that the strategy performed poorly on this dataset during the given timeframe. This emphasizes the importance of thorough backtesting and strategy evaluation before actual deployment.
To understand the strategy's behavior, let's visualize the stock price, the Bollinger Bands (Middle, Upper, and Lower bands), and the buy/sell points.
The graph illustrates the stock's closing price (in blue), the middle Bollinger Band (20-day SMA in green), the upper Bollinger Band (in red), and the lower Bollinger Band (in cyan). Buy signals are denoted by green upward-pointing triangles, while sell signals are shown as red downward-pointing triangles.
Observations:
As a Stock Analyst focusing on momentum strategies, compile a detailed report on APPLE stock using the Rate of Change (ROC) as an indicator. Investigate periods where the ROC indicates significant positive or negative momentum, suggesting potential buying or selling opportunities, respectively. Backtest the ROC signals against historical data to validate the strategy's robustness. Present your conclusions using charts that clearly display ROC values alongside stock price movements.
Response: Momentum strategies seek to capitalize on the continuation of existing trends in the market. The underlying idea is that assets that have performed well in the past are likely to continue performing well, and vice versa.
Momentum Strategy using Rate of Change (ROC):
The Rate of Change (ROC) is a momentum oscillator that measures the percentage change in price from one period to the next. The ROC can be used to identify the direction of the trend or gauge its strength.
Trading Rules:
1. Buy Signal: When the ROC crosses above a predefined threshold (e.g., 0 or another positive value), indicating positive momentum.
2. Sell Signal: When the ROC crosses below a predefined threshold (e.g., 0 or another negative value), suggesting negative momentum.
Steps:
1. Compute the ROC for a specified period (e.g., 14 days).
2. Generate buy/sell signals based on the ROC crossing predefined thresholds.
3. Backtest the strategy using historical data.
Let's implement and backtest this momentum strategy on the provided dataset. After backtesting the momentum strategy using the Rate of Change (ROC) on the dataset:
The outcome suggests that the strategy did not perform well on this dataset during the given timeframe. The chosen threshold or the ROC period might not have been optimal for this specific stock.
To gain a better understanding, let's visualize the stock price, the ROC values, and the buy/sell points.
The visualization provides insights into the momentum strategy using the Rate of Change (ROC):
1. The top graph displays the stock's closing price (in blue). Buy signals are shown with green upward-pointing triangles, while sell signals are denoted by red downward-pointing triangles.
2. The bottom graph visualizes the ROC values (in purple) with a black zero line for reference.
Observations:
As a Stock Analyst specializing in breakout trading strategies, prepare a comprehensive report on APPLE stock. Identify periods where the stock price breaks out from a defined range, either reaching new highs or lows, hinting at a potential prolonged move in the breakout direction. Incorporate technical indicators like volume or relative strength to validate the strength of the breakout. Backtest identified breakout signals against historical data to evaluate the strategy's consistency. Present your findings with charts illustrating breakout points and accompanying volume or indicator data.
Response: Breakout trading is a strategy where traders aim to enter the market when the price makes a breakout from a previously defined resistance or support level. The rationale behind this strategy is that when a significant resistance or support level is broken, it's likely a large number of traders will enter or exit their positions, leading to a sharp price movement.
Breakout Trading Strategy:
Trading Rules:
To implement this strategy, we'll need to define our resistance and support levels. One common method is to use recent highs as resistance and recent lows as support. For our implementation, we'll use:
For this demonstration, we'll use \( n = 14 \) (a 14-day window) to define our resistance and support levels. After determining these levels, we'll generate buy/sell signals based on price breakouts and then backtest the strategy.
Let's implement and backtest the breakout trading strategy on the provided dataset.
It seems there was an error due to non-numeric data types in the "High" and "Low" columns. Let's first clean up the "High" and "Low" columns by converting them to float, and then we'll proceed with the breakout trading strategy.
After backtesting the breakout trading strategy on the dataset:
The breakout strategy is contingent on significant price movements that breach the defined resistance or support levels. It's possible that within the dataset's timeframe, there weren't any significant breakouts that met the strategy's criteria, or the breakout window (14 days) chosen was not optimal.
To better understand the strategy's behavior, let's visualize the stock price, resistance, support levels, and the buy/sell points.
The graph showcases the stock's closing price (in blue), the resistance level (in red), and the support level (in green). Buy signals are represented with green upward-pointing triangles, while sell signals are shown as red downward-pointing triangles.
Observations:
As a Stock Analyst with a focus on technical strategies, draft an in-depth analysis of APPLE stock employing the moving average crossover technique. Concentrate on instances where a short-term moving average (e.g., 50-day) intersects a long-term moving average (e.g., 200-day), signaling potential buy or sell opportunities. For added clarity, chart these moving averages on APPLE's stock price graph, marking crossover points. Backtest observed crossover events against past data to determine the strategy's reliability and effectiveness. Conclude with a visualization that combines stock prices with moving average lines, emphasizing key crossover events.
Response: The Moving Average Crossover strategy is one of the most widely recognized techniques in technical analysis. It involves two moving averages: one representing a short period and the other a longer period.
Moving Average Crossovers Strategy:
1. Short-term Moving Average: A moving average of a shorter period (e.g., 50 days).
2. Long-term Moving Average: A moving average of a longer period (e.g., 200 days).
Trading Rules:
1. Buy Signal (Golden Cross): When the short-term moving average crosses above the long-term moving average.
2. Sell Signal (Death Cross): When the short-term moving average crosses below the long-term moving average.
For this demonstration, we'll use:
We'll calculate these moving averages, identify the crossover points, and backtest the strategy on the provided dataset.
Using the Moving Average Crossover strategy:
This outcome suggests that there weren't any significant crossovers between the 50-day and 200-day moving averages during the dataset's timeframe. To better understand the strategy's behavior, let's visualize the stock price, the two moving averages, and any potential crossover points.
The graph showcases the stock's closing price (in blue), the 50-day moving average (in green), and the 200-day moving average (in red). Golden Crosses (potential buy signals) are represented with green upward-pointing triangles, while Death Crosses (potential sell signals) are shown as red downward-pointing triangles.
Observations:
So, after navigating the vast sea of stock trading, it's evident that people have a variety of ways to approach their decisions. From sailing with the direction of the market to waiting patiently for those significant shifts, everyone's got their own strategy. It's a bit like choosing between hiking trails; some prefer the scenic route while others opt for the straightforward path. And with the help of modern tools like CHATGPT's data analysis, making these choices becomes a tad simpler. It's like having a handy guidebook for a complex journey. By understanding these methods and using available resources, anyone can navigate the stock market more confidently. It's all about finding what works best for you and sticking to it.
Dr. Anshul Saxena is an author, corporate consultant, inventor, and educator who assists clients in finding financial solutions using quantum computing and generative AI. He has filed over three Indian patents and has been granted an Australian Innovation Patent. Anshul is the author of two best-selling books in the realm of HR Analytics and Quantum Computing (Packt Publications). He has been instrumental in setting up new-age specializations like decision sciences and business analytics in multiple business schools across India. Currently, he is working as Assistant Professor and Coordinator – Center for Emerging Business Technologies at CHRIST (Deemed to be University), Pune Lavasa Campus. Dr. Anshul has also worked with reputed companies like IBM as a curriculum designer and trainer and has been instrumental in training 1000+ academicians and working professionals from universities and corporate houses like UPES, CRMIT, and NITTE Mangalore, Vishwakarma University, Pune & Kaziranga University, and KPMG, IBM, Altran, TCS, Metro CASH & Carry, HPCL & IOC. With a work experience of 5 years in the domain of financial risk analytics with TCS and Northern Trust, Dr. Anshul has guided master's students in creating projects on emerging business technologies, which have resulted in 8+ Scopus-indexed papers. Dr. Anshul holds a PhD in Applied AI (Management), an MBA in Finance, and a BSc in Chemistry. He possesses multiple certificates in the field of Generative AI and Quantum Computing from organizations like SAS, IBM, IISC, Harvard, and BIMTECH.
Author of the book: Financial Modeling Using Quantum Computing