Understanding RL-based NAS
RL is a family of learning algorithms that deal with the learning of a policy that allows an agent to make consecutive decisions on its actions while interacting with states in an environment. Figure 7.3 shows a general overview of RL algorithms:
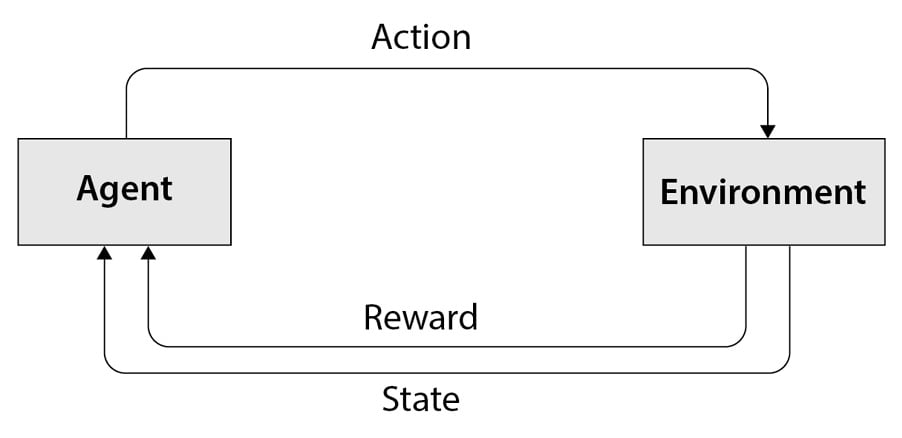
Figure 7.3 – General overview of RL algorithms
This line of algorithms is most popularly utilized to create intelligent bots for games that can act as offline players against real humans. In the context of a digital game, the environment represents the entire setting in which the agent operates, including aspects such as the position and status of the in-game character, as well as conditions of the in-game world. The state, on the other hand, is a snapshot of the environment at a given time, reflecting the current conditions of the game. One key component in RL is the environment feedback component that can provide either a reward or punishment. In digital games, examples of rewards...